Why is Artificial Intelligence so popular?
FAQ|Why is Artificial Intelligence so popular?AI (Artificial Intelligence) is a science and technology that enables machines to mimic human intelligence. Through software, algorithms, and data, it allows machines to possess perception, learning, reasoning, and decision-making abilities similar to those of humans.
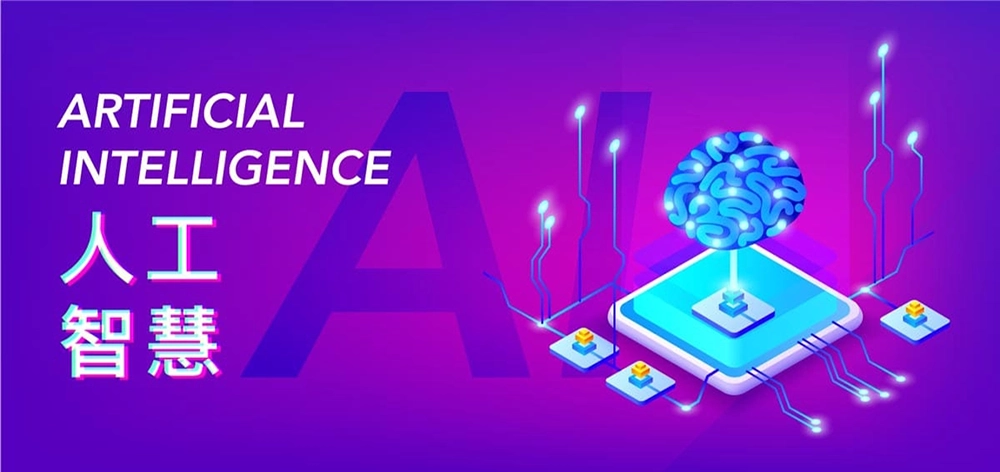
Edge computing
The Bridge to Smart Manufacturing: Edge Computing, IoT, and AI
AI Artificial Intelligence Brings Convenience to Life
AI brings convenience to people’s lives and extends its applications to various research fields, such as smart cities, smart agriculture, smart transportation, smart manufacturing, and smart homes. But what exactly is AI? And what are its core capabilities?
AI (Artificial Intelligence) is a science and technology that enables machines to mimic human intelligence. Through software, algorithms, and data, it allows machines to have perception, learning, reasoning, and decision-making abilities similar to humans. The goal of AI is to create systems that can perform specific tasks, provide advice, or autonomously solve problems.
Core Abilities of Artificial Intelligence (AI)
Five Key Core Capabilities
AI (Artificial Intelligence) is gradually integrating into our daily lives, from voice assistants to autonomous vehicles, with applications across various industries. Behind these advanced technologies is a set of core capabilities that enable machines to mimic or even surpass human intelligence. Below are the five core capabilities of AI:
1. Perception
Perception is one of AI’s foundational abilities, referring to a machine’s ability to understand various information from the outside world. This information may come from vision (images), hearing (sound), language, or other environmental data. Through perception, AI can “see” and “hear” the world, transforming this data into something understandable. For example, facial recognition technology helps machines identify individuals, while voice recognition systems like Siri or Google Assistant understand spoken commands and respond accordingly. To enable AI to “see” and “hear,” various data collection devices or sensors, such as cameras, microphones, and sensors, are used.
2. Reasoning
Reasoning enables AI to logically analyze existing data to draw conclusions or create action plans. This is a key aspect of AI’s intelligent operation, as it not only accepts information but also makes rational inferences based on that data. This also requires engineers to use specialized algorithms to analyze the data and AI to assist with accelerating the computation process. For example, the AlphaGo program used its strong reasoning ability to analyze the board situation and predict the opponent’s moves, ultimately defeating a world-class Go player.
3. Learning
Learning is the driving force behind machine intelligence’s continuous evolution. By learning, accumulating, and cleaning data, AI can extract patterns from past experiences and data, using those patterns to improve future performance. This is the core concept of machine learning, where AI improves through ongoing practice. Many applications, like Netflix or Spotify’s recommendation systems, rely on this learning ability by analyzing users’ historical behavior to provide personalized content recommendations.
4. Decision Making
AI’s decision-making ability allows it to choose the best course of action based on the analysis results. This ability is crucial across many fields, especially in fast-changing environments. For example, autonomous vehicles rely on their decision systems to plan the driving path based on road conditions, traffic rules, and pedestrian positions, ensuring driving safety and efficiency.
5. Natural Interaction
Natural interaction is the ability for AI to communicate with humans, not only including understanding and generating human language but also involving intelligent behavioral responses. With advancements in technology, AI can interact more naturally with humans, providing a smooth conversational experience. For example, smart customer service chatbots understand and respond to customer questions, not only offering answers but also engaging in deeper conversations to enhance user experience.
Conclusion
In summary, AI’s core capabilities encompass Perception, Reasoning, Learning, Decision Making, and Natural Interaction. These abilities enable AI to function effectively in many complex scenarios, accelerating our move toward an intelligent future. As these technologies continue to advance, AI will change human lifestyles in even more fields and have a broader impact.
Main Types of Artificial Intelligence (AI)?
Types and Key Technologies
AI, full of infinite possibilities, can be divided into three major types based on its level of intelligence and application range: Weak AI, Strong AI, and Super AI. In addition, the development of AI depends on several key technologies, such as machine learning, deep learning, natural language processing, computer vision, and reinforcement learning.
1. Weak AI (Narrow AI)
Weak AI focuses on performing specific tasks and can only operate within its designed scope. It cannot exceed its defined functions and does not possess self-learning capabilities. This type of AI has been widely applied in real life and has shown excellent performance in many fields. Common examples include voice assistants (such as Siri, Google Assistant) and medical image analysis systems. Although these systems appear to be intelligent, their abilities are limited, and they cannot multitask like humans.
2. Strong AI (General AI)
Strong AI refers to systems with intelligence comparable to that of humans, capable of handling various different tasks. This type of AI possesses self-learning and adaptive abilities, adjusting based on environment and experience, and can perform excellently in multiple domains. However, Strong AI is still in the research phase and has not been realized yet. The ultimate goal is to create an intelligent system that can understand and learn like humans, with capabilities in reasoning, planning, language understanding, and more.
3. Super AI (Superintelligence)
Super AI refers to an intelligence system that surpasses human intelligence in all areas, including creativity, emotional intelligence, and solving complex problems. Such AI can not only perform tasks that humans cannot accomplish but can also exhibit innovation and efficiency in problem-solving that humans cannot match. Super AI currently only exists in science fiction and has not been achieved yet, but it remains a central concept in humanity’s imagination and exploration of the future development of AI.
Type | Definition | Examples | Status |
---|---|---|---|
Weak AI | Focused on specific tasks, cannot exceed its design scope. | Voice assistants, recommendation systems, medical image analysis | Implemented |
Strong AI | Possesses human-level intelligence, capable of performing various tasks. | None (Currently a research goal) | In research |
Super AI | Intelligence surpasses human capabilities in all fields, including creativity and emotional intelligence. | None (Only exists in science fiction) | Not yet realized |
Key AI Technologies
The realization of AI depends on several key technologies that enable machines to understand, learn, and perform complex tasks.
1. Machine Learning (ML)
Machine learning allows computer systems to learn from data without relying on preset program instructions. By learning from large datasets, machines can discover patterns and improve their performance. ML is widely used in various fields, including financial risk assessment and personalized recommendations.
2. Deep Learning (DL)
Deep learning is a branch of machine learning that uses multi-layered neural networks to mimic the structure of the human brain and process large amounts of high-dimensional data. This technology has achieved significant success in areas such as image recognition and speech processing. For instance, deep learning is used in facial recognition, voice assistants, and autonomous vehicles.
3. Natural Language Processing (NLP)
NLP is the technology that enables machines to understand, generate, and respond to human language. This technology allows machines to perform speech recognition, language translation, sentiment analysis, and more. Applications like Google Translate and chatbots are based on NLP.
4. Computer Vision
Computer vision allows machines to “see” and interpret visual information, similar to the human visual perception system. This technology is widely used in image recognition, object detection, facial recognition, and more. It forms the basis for advanced technologies like autonomous vehicles and medical image analysis.
5. Reinforcement Learning (RL)
Reinforcement learning is a type of machine learning where machines learn how to achieve specific goals through interaction with their environment. Machines adjust their behavior through feedback signals to optimize strategies, ultimately achieving the best action plan. RL has been applied in games, robotics, and financial investment.
6. Speech Technology
Speech technology enables AI to process speech signals, including speech recognition and synthesis. It involves speech-to-text (STT) and text-to-speech (TTS) systems. Deep learning further enhances the naturalness of speech generation. For example, Siri, Google Assistant, voice navigation for the visually impaired, and smart home voice-activated devices all rely on speech technology.
7. Generative AI
Generative AI creates new content, such as images, text, or music, by learning data patterns. This technology is based on Generative Adversarial Networks (GAN) or Variational Autoencoders (VAE). Recent models like GPT-4 and Stable Diffusion have enhanced various applications, including generating images, animations, music, virtual hosts, and virtual idols.
8. Edge AI
Edge AI involves deploying AI computation on various edge devices (such as smartphones, cameras, and industrial computers). It provides low-latency, high-privacy local computation that does not rely on the cloud, making it suitable for real-time applications. Applications include predictive maintenance systems for devices, real-time health data analysis for wearable devices, and voice control/data processing in smart homes.
9. Decision Systems
Decision systems provide the best recommendations for business or operations based on data and models, applying data science and machine learning techniques, including simulation and optimization algorithms. Examples include path optimization for large logistics companies, inventory forecasting, clinical decision support systems in medical technologies, and pricing strategies/consumer behavior analysis in market research.
Technology Name | Definition | Application Range |
---|---|---|
Machine Learning | Enables machines to learn and improve from data without relying on preset instructions. | Data analysis, recommendation systems, financial risk assessment |
Deep Learning | Uses multi-layered neural networks to mimic the structure of the human brain and process high-dimensional data. | Image recognition, speech processing, autonomous driving |
Natural Language Processing | Enables machines to understand, generate, and respond to human language. | Chatbots, voice assistants, language translation |
Computer Vision | Gives machines the ability to “see,” performing image recognition and object detection. | Autonomous driving, medical image analysis |
Reinforcement Learning | Enables machines to learn how to achieve specific goals through interaction. | Games, robot control, financial investment |
Speech Technology | Deep learning enhances the naturalness of speech generation. | Siri, voice navigation, smart home devices |
Generative AI | Learns data patterns to create new content. | Image generation, animations, music, virtual hosts |
Edge AI | Deploys computations on edge devices, suitable for real-time applications. | Predictive systems, wearable devices |
Decision Systems | Integrates data science and machine learning techniques, involving simulation and optimization algorithms. | Logistics path optimization, clinical decision-making, consumer behavior analysis |
Extended AI Technologies
Understanding LLM and LMM
Two Key Concepts in Artificial Intelligence and Statistics
In the development of modern science and technology, two terms, LLM and LMM, play crucial roles in the fields of artificial intelligence and statistics. Although their names are similar, the concepts and application areas they represent are vastly different. This article will provide a detailed explanation of these two terms to help readers better understand their background and uses.
LLM (Large Language Model): Core Technology for Natural Language Processing
LLM (Large Language Model) represents a major breakthrough in the field of artificial intelligence, particularly in natural language processing (NLP). Large language models use deep learning techniques to understand, generate, and process natural language. These models are trained on vast amounts of text data, learning to recognize patterns and structures in language, thus enabling them to generate and understand language.
Core Features of LLM include:
Language Understanding and Generation: LLM can understand the syntax and semantics of sentences and generate logically coherent responses. This allows them to perform various language tasks such as automated answering, article writing, sentiment analysis, etc.
Large-Scale Training Data: LLMs are typically trained on billions of pieces of text data, sourced from books, articles, web pages, and other materials, which helps capture various layers of language.
Pre-training and Fine-Tuning: These models are first pre-trained on a large corpus of data and then fine-tuned for specific applications. For example, GPT models are trained in this manner and excel at various language generation tasks.
Currently, LLMs like GPT-3 and GPT-4 have achieved significant accomplishments in language generation, machine translation, dialogue systems, and more. They are capable of simulating human language understanding and expression, making a significant impact in various intelligent applications.
LMM (Linear Mixed Model): A Statistical :Tool for Handling Complex Data Structures
In contrast to LLM, LMM (Linear Mixed Model) is a commonly used model in statistics, primarily applied to analyze data with hierarchical structures or repeated measurements. LMM can simultaneously account for both fixed and random effects, making it suitable for various situations involving random variables.
Key Features of LMM:
Fixed and Random Effects:LMM not only handles fixed effects (i.e., effects that are the same for all observations) but also considers random effects (i.e., effects that differ across observations). This makes LMM especially well-suited for dealing with complex structured data.
Adaptability to Repeated Measurement Data: LMM is an ideal tool for dealing with repeated measurement data. For example, when conducting multiple tests on the same patient, LMM can account for differences between patients and the variability in measurements taken at different times.
Hierarchical Structure Analysis: LMM can handle hierarchical structures in data, which is particularly important in fields like social science and biology. For example, when analyzing students’ academic performance, LMM can account for the influence of the schools they attend.
LMM is widely used in fields such as biology, medicine, psychology, and other areas that deal with data involving multiple measurements and hierarchical structures. It plays a critical role in helping researchers interpret the variability in data more accurately, leading to more precise conclusions.
Summary
While LLM and LMM share similar names, they belong to entirely different fields and serve different purposes. LLM is a core concept in artificial intelligence, driving the development of natural language processing technologies, and is applied in various language generation and understanding tasks. In contrast, LMM is a statistical tool used for handling complex data that includes both fixed and random effects, and it is widely applied in research that involves multiple measurements and hierarchical analysis.
Extended AI Technologies
AI-Driven Innovative Algorithms and Applications
These new algorithms expand the application scenarios of AI, ranging from image generation and natural language processing to multimodal data processing. In the future, more innovative technologies that combine different architectures may emerge. These technologies not only drive industry upgrades but also provide new ideas to solve social problems.
1. Transformers and Derivative Models
Transformer is a deep learning architecture introduced by Google in 2017, which revolutionized sequence data processing through the “Attention Mechanism.” It is particularly suitable for Natural Language Processing (NLP). It not only enhances text understanding and generation but also has made waves in other fields.
Derivative Model | Function | Main Applications |
---|---|---|
BERT | Bidirectional semantic understanding, suitable for NLP tasks | Sentiment analysis, semantic search, machine translation |
GPT | Text generation, emphasizes generative ability | ChatGPT dialogue generation, text creation |
ViT | Deep learning tool for image processing | Image classification, object detection |
Perceiver | A general model for multimodal data processing | Unified processing of sound, images, and text |
2. Diffusion Models
Diffusion models are generative AI models that generate high-quality data by adding noise and denoising. They outperform Generative Adversarial Networks (GAN) in terms of stability and detail handling.
Derivative Model | Function | Main Applications |
---|---|---|
DALL·E 2 | Text-to-image generation | Digital creation, design assistance |
Stable Diffusion | Lightweight image generation model | Artistic style image generation, material design |
Imagen | High-resolution image generation model | Advertising design, animation production |
3. Advances in Reinforcement Learning (RL)
Reinforcement learning (RL), combined with deep learning techniques, allows models to learn the optimal strategies by interacting with the environment. Recent developments focus on multi-agent systems, imitation learning, and long-term planning.
Derivative Model | Function | Main Applications |
---|---|---|
AlphaZero | Self-play to learn the best strategies | Game design, automated decision-making |
MuZero | No need for predefined rules, more adaptable | Dynamic environment optimization (e.g., logistics path planning) |
Soft Actor-Critic (SAC) | Improves the stability of continuous control problems | Robot control, autonomous driving |
4. Few-Shot Learning & Zero-Shot Learning
These technologies focus on solving the “data scarcity” problem, enabling models to make accurate predictions even with minimal or no training data.
Derivative Model | Function | Main Applications |
---|---|---|
CLIP | Combines text and image multimodal learning | Image retrieval, text-image matching |
GPT-3 | Supports few-shot and zero-shot learning | Multilingual translation, question answering |
5. Graph Neural Networks (GNNs)
GNNs are designed to process graph-structured data, efficiently modeling the relationships between nodes, edges, and graphs, showing great potential in fields like social networks and knowledge graphs.
Derivative Model | Function | Main Applications |
---|---|---|
GAT | Uses attention mechanism to enhance modeling ability | Social network analysis, knowledge graph construction |
Molecular Graph Networks | Models molecular structures | Drug development, material design |
6. Self-Supervised Learning
Self-supervised learning makes full use of unlabeled data, reducing reliance on manual annotations while learning efficient feature representations.
Derivative Model | Function | Main Applications |
---|---|---|
SimCLR | Contrastive learning for image feature extraction | mage classification, object detection |
BYOL | Simplifies contrastive learning, no need for negative samples | Representation learning, unsupervised learning |
7. New Generative Models
The advancements in generative models have brought high-quality generation results, expanding applications to content creation, game design, etc.
Derivative Model | Function | Main Applications |
---|---|---|
StyleGAN | High-quality multi-style image generation | Game character design, virtual idol creation |
VQ-VAE-2 | Detailed image and video generation | Content creation, video compression |
8. Neuro-Symbolic AI
Neuro-symbolic AI combines symbolic reasoning with deep learning, enhancing the interpretability and reasoning capabilities of AI systems, especially in data-sparse scenarios.
Derivative Model | Function | Main Applications |
---|---|---|
DeepProbLog | Combines probabilistic logic and neural networks | Logical reasoning, knowledge graph construction |
NS-CL | Combines symbolic representation and deep learning | Image question answering, educational reasoning |