Proprietary Technology & Philosophy
Home|AboutThrough GoodTech’s exclusive proprietary algorithms, automated analysis and statistical processing enable autonomous decision-making and even predictive outcomes. By optimizing system operation processes, we provide truly practical and user-friendly solutions, empowering users to make the most accurate decisions for their production line equipment.
Four Key Advantages of GoodTech Monitoring Solutions
Low Technical Barrier
The system comes with built-in ISO standards commonly used for rotors, such as ISO 10816 and 2372. Additionally, GoodTech has taken the lead in the industry by incorporating the ISO 20816 standard, providing a more comprehensive management solution for rotor equipment.
With these pre-installed standards, there’s no need for users to memorize tables or configure complex technical settings. Simply select the corresponding power and speed to start measurements, making the system suitable for users without a professional background.
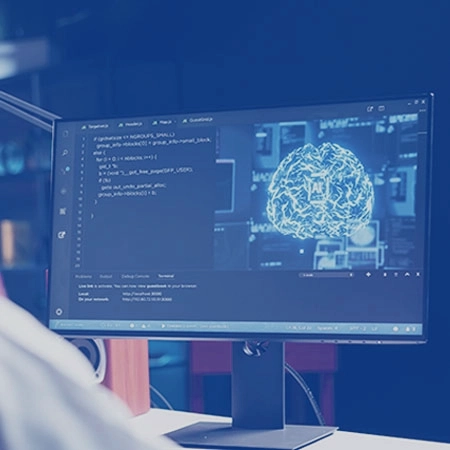
Measurement Algorithm Development
GoodTech specializes in developing measurement algorithms to provide precise data analysis and practical solutions. Our proprietary algorithms automate data analysis and statistical calculations, enabling autonomous decision-making and even predictive results.
By optimizing system operations, we offer truly user-friendly and effective solutions to help users make the most accurate decisions for production line equipment.
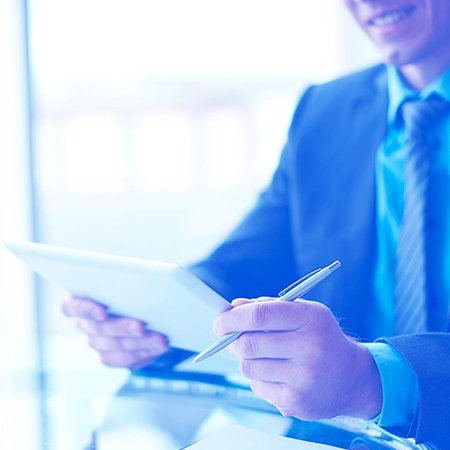
Flexible Software and Hardware Expansion
GoodTech’s software and hardware systems are designed with maximum flexibility. The software architecture allows for scalable expansion, while the hardware provides options that users can customize based on factory environment conditions and equipment monitoring requirements.
GoodTech understands that system implementation cannot always be completed at once, so we prioritize mechanisms that allow for convenient future expansion, ensuring long-term adaptability and scalability.
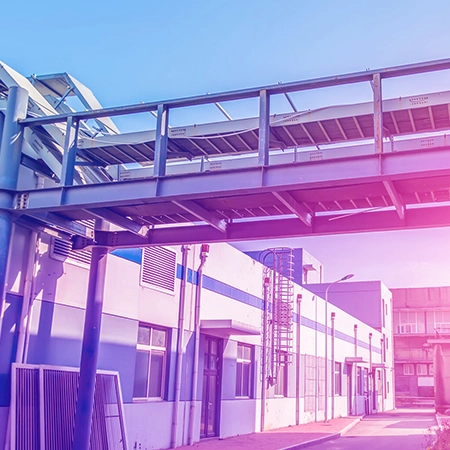
Fastest Benefits
GoodTech’s monitoring system primarily adopts non-intrusive measurement methods, allowing plug-and-play functionality. Users can see immediate benefits and start managing equipment as soon as the system is installed.
Unlike traditional AI systems that require extensive data accumulation over time, GoodTech’s machine learning intelligent monitoring system provides instant value upon deployment, delivering immediate insights and operational improvements.
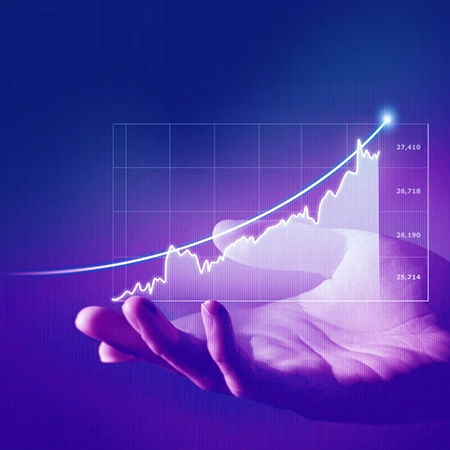
Three Core Technologies
Based on Rule-Based principles and proprietary algorithms, GoodTech creates the best value for customers.
1. Suitable for Rotor Equipment
SOA Algorithm – 4 Times Faster Benefits
Optimized for rotor equipment, the SOA (Signal-Oriented Analysis) algorithm significantly accelerates performance analysis, delivering benefits up to four times faster than traditional methods.
2. Edge Computing with EDGE-IOT
See What You Want Without Recalculation
Our EDGE-IOT technology enables real-time data processing at the edge, eliminating the need for redundant calculations and allowing users to access critical insights instantly.
AI Smart Monitoring in Rotor Equipment Management
With the rapid development of smart manufacturing, the reliability and stability of equipment operations have become critical pillars of business competitiveness. Particularly for rotor equipment, which is widely used in industries like semiconductors, petrochemicals, and power generation, its operational status directly impacts production efficiency and safety.
However, traditional equipment monitoring methods, such as fixed schedule maintenance or ISO vibration standard monitoring, often suffer from limited data and insufficient early anomaly detection, leading to excessive maintenance or undetected faults. To address these challenges, GoodTech Technology combines Artificial Intelligence (AI), Predictive Maintenance (PHM), and Reliability-Centered Maintenance (RCM) to develop an industry-leading monitoring solution for rotating equipment.
Application Overview
This AI monitoring model captures 25 characteristic values through wireless sensors and evaluates the equipment’s health status based on ISO standards’ four levels: Excellent, Normal, Warning, and Danger. The core functions of the AI include:
1. Health Status Assessment:
Analyzes the probability of the equipment’s operational status based on characteristic value combinations.
2. Trend Monitoring:
Displays each monitoring result with color-coded lights to help identify abnormal or deteriorating trends.
3. Preventive Suggestions:
Provides maintenance or servicing recommendations to reduce downtime risk when warning or danger states are detected.
Technical Overview
Currently, this AI-based monitoring model has been pre-trained by GoodTech Technology, using data from 25 characteristic values collected by wireless sensors. The AI prediction focuses on the quality status levels of rotating equipment, which are categorized in four levels: Excellent, Normal, Warning, and Danger, following ISO standards.
The trend chart shown in the AI model represents the characteristic values sent by the sensors, which are converted into the “probability” of the equipment’s state. By observing the proportion of colored status blocks, users can determine the current health of the equipment at any given point.
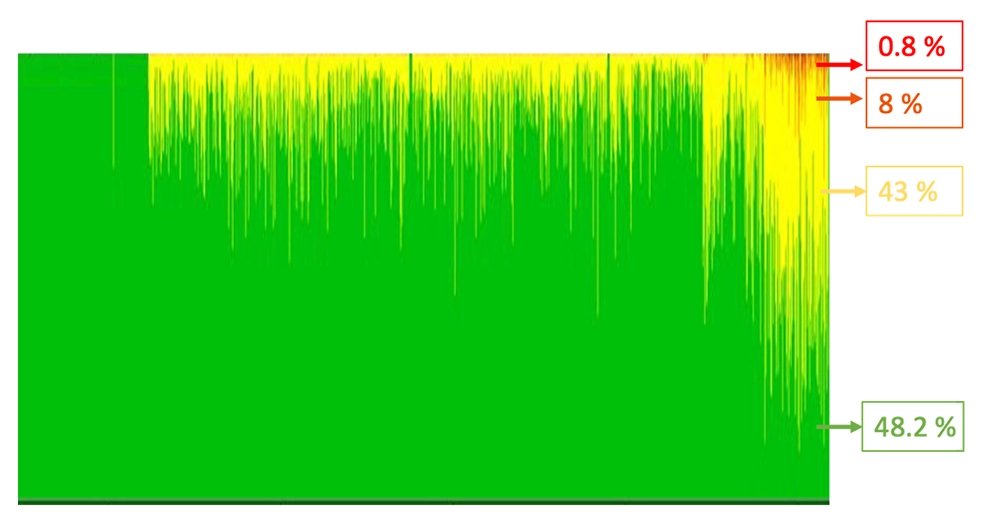
Example of AI Results: As shown in the example above, the AI results suggest that the equipment has a 91.2% probability of being in normal operation.
In the Y-direction vibration values, applying ISO vibration standards showed a warning (orange light), which means maintenance should be scheduled. However, when other vibration characteristic values are added and predicted by the AI model, the equipment is determined to be in a Normal-Excellent state, meaning it is still capable of functioning properly.
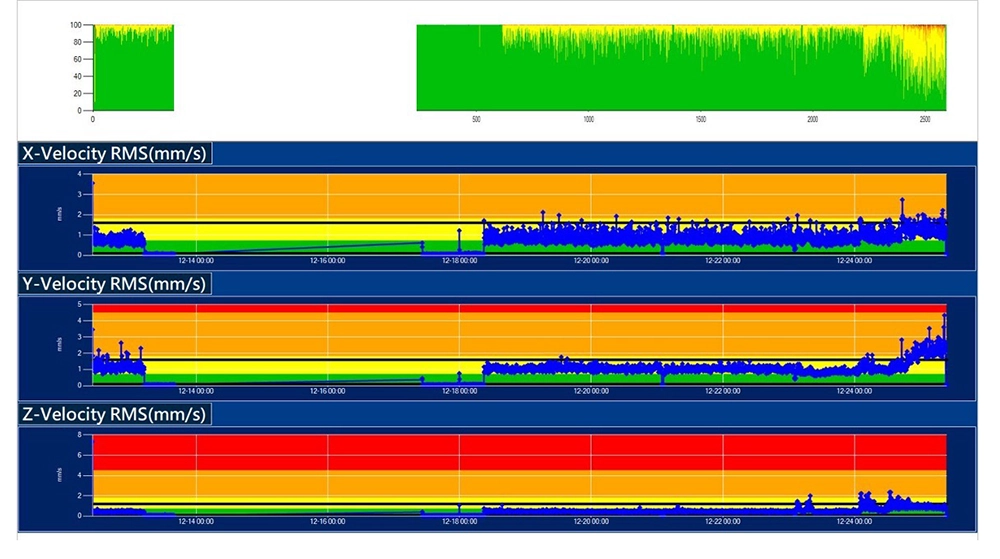
Apply ISO vibration regulations and display as warning
ISO Vibration Standard vs. AI Monitoring
The above comparison demonstrates that the ISO vibration standard may be stricter, but it is not necessarily inaccurate. Historically, the equipment monitored by the ISO vibration standard was used mainly in industries like petrochemicals, power generation, and nuclear plants, where equipment is highly valuable, and failure without warning can lead to safety hazards. Therefore, experts and researchers have defined stricter standards based on extensive big data analysis of rotor equipment.
This approach aligns with the goals and principles of predictive maintenance. Comparing both management methods, it’s evident that when ISO vibration limits are exceeded, the AI quality status trend also shows a deterioration, reinforcing the importance of AI optimization.
AI-optimized monitoring
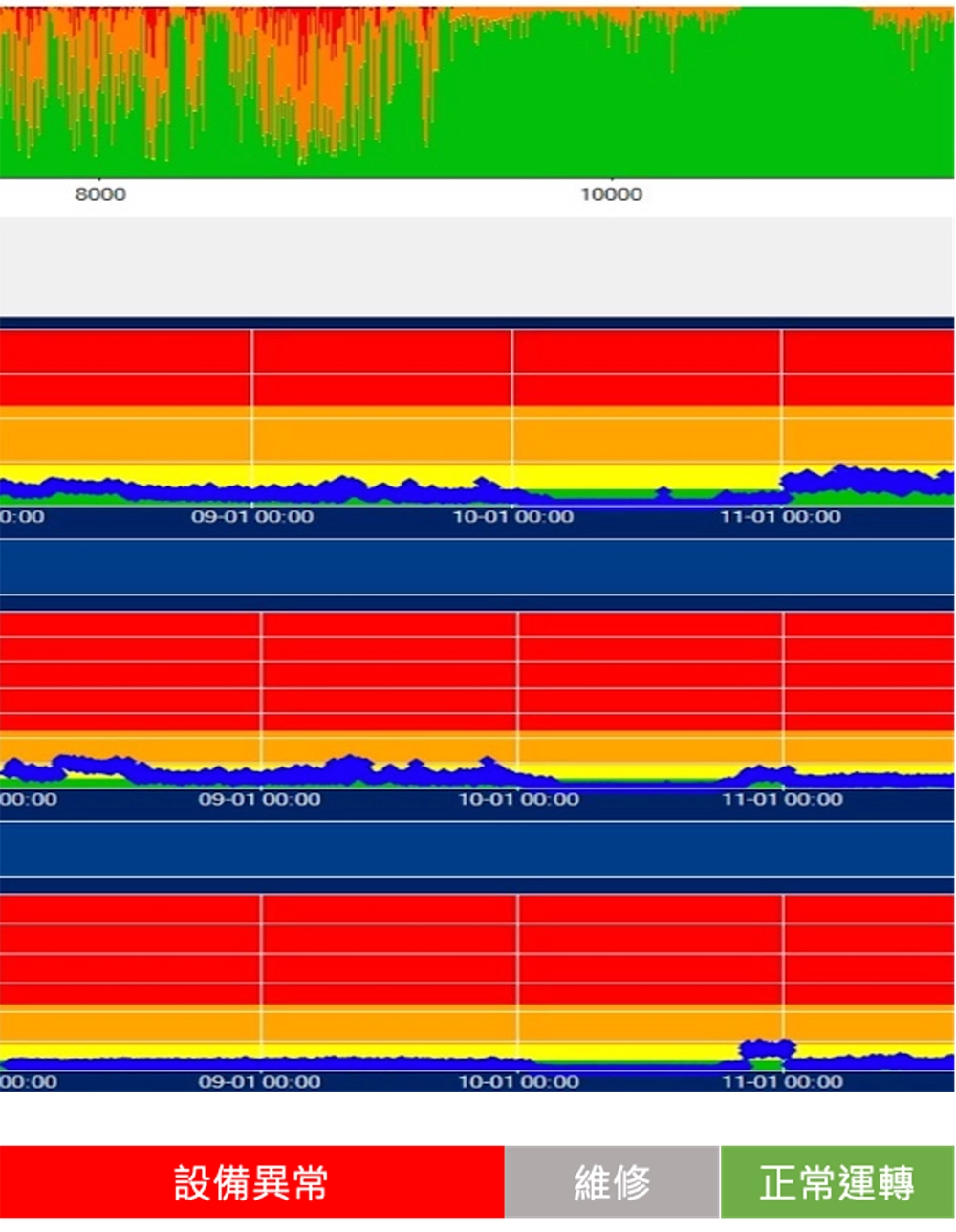
The image above shows another example of AI-optimized monitoring. Comparing the two monitoring models, if damage to a machine cannot be detected via vibration velocity, AI’s optimized monitoring model can identify abnormal states. In this case, the AI detected a Warning-Danger state in the equipment. After a month of maintenance and re-commissioning, even though the vibration values showed no significant changes, the AI model detected the machine in an Excellent state after repairs.
This case clearly demonstrates that as computer capabilities grow, adding more vibration features and calculation mechanisms and appropriately designing AI monitoring models can enhance previously defined vibration standards. This leads to better early anomaly detection and improved predictive capabilities.
Technical Advantages
1. Wide Applicability: Unlike the ISO standard which relies on a single vibration metric, the AI model can analyze more characteristics, offering broader applicability.
2. Accurate Prediction: The AI model can detect abnormalities earlier than ISO, preventing delayed maintenance.
3. Dynamic Adjustment: The AI model optimizes algorithms according to different industrial scenarios, meeting diverse needs.
4. Continuous Optimization: As data increases and models are updated, the prediction accuracy improves over time.
Features
1. Multi-Feature Value Integration:
GoodTech’s AI monitoring model uses 25 feature values collected from sensors, covering data such as vibration speed, acceleration, and temperature. Compared to traditional methods that rely on a single vibration value (ISO standard), GoodTech’s model greatly improves the accuracy of equipment health status assessments.
2. Combining ISO Standards with AI:
GoodTech’s model uses ISO vibration standards to classify equipment status (Excellent, Normal, Warning, Danger), further optimized by AI. This combination allows the AI model to detect early abnormal trends even when ISO values meet the standard, enabling more refined management.
3. Health Status Trend Prediction and Visualization:
GoodTech’s system uses color-coded lights to display the distribution and changes in health status, helping users quickly assess the equipment’s condition. The trend analysis feature allows users to plan maintenance in advance and reduce the risk of unplanned downtime.
4. Optimized Abnormality Detection:
Traditional methods struggle to detect early anomalies (such as slight changes in characteristic values). The AI model enhances anomaly detection sensitivity, especially when analyzing multiple features. This capability is particularly important for critical equipment like semiconductor manufacturing machines or petrochemical compressors, effectively preventing major failures.
5. Dynamic Learning and Model Optimization:
GoodTech’s AI model has continuous learning capabilities, optimizing its prediction accuracy as new data is collected from actual applications. Over time, the model adapts to specific equipment operational needs.
6. Wide Applicability:
GoodTech’s technology is applicable in multiple industrial scenarios, including semiconductors, petrochemicals, power generation, and stamping equipment, showcasing its flexibility and adaptability.
Technical Differentiation
GoodTech’s AI-powered monitoring technology integrates multi-feature data, deep learning, and ISO standard optimization, making significant breakthroughs in equipment health management. Compared to traditional methods, GoodTech’s technology improves anomaly detection accuracy and seamlessly combines predictive maintenance and reliability-centered maintenance, offering customers a more cost-effective, efficient, and flexible solution.
Technical Differences | GoodTech | Traditional Technology |
---|---|---|
1. Data Processing Depth & Scope | Utilizes up to 25 feature values with AI for deep data processing, capturing more comprehensive equipment status information.Win | Primarily relies on a single vibration data point, such as vibration speed, for health status assessment, with limited analytical range. |
2. Anomaly Detection Accuracy | Personalized health state modeling with AI, detecting early anomalies that ISO standards miss, preventing risks.Win | Typically relies on fixed standards (e.g., ISO), but lacks the ability to detect personalized anomalies specific to equipment. |
3. Trend Analysis & Predictive Capability | Predicts health status changes in advance through trend analysis, supporting proactive maintenance planning. Win | Primarily static monitoring, diagnosing only after issues occur, lacking foresight. |
4. Model Dynamic Optimization | Dynamic learning ability that continuously evolves the model as new data accumulates, adapting to diverse needs. Win | Static standards that don’t adapt in real-time based on new data. |
5. Visualization & User Experience | Provides intuitive visual health status charts, reducing the technical barrier for users to understand equipment condition.Win | Simpler health status indicators, requiring interpretation by professionals. |
6. Economic Benefits & Practicality | Significant reduction in maintenance costs and improved production efficiency through intelligent monitoring and accurate maintenance suggestions.Win | Over-reliance on expert experience, leading to higher costs and limited efficiency. |
PHM and RCM in Rotor Equipment Management
With the rapid development of smart manufacturing, the reliability and stability of equipment operations have become crucial pillars for business competitiveness. Rotor equipment, in particular, is widely used in industries such as semiconductors, petrochemicals, and power generation, where its operational status directly affects production efficiency and safety.
However, traditional equipment monitoring methods such as fixed-schedule maintenance or ISO vibration standard monitoring often suffer from single data sources and limited early anomaly detection, leading to excessive maintenance or undetected faults. To tackle these challenges, GoodTech Technology integrates Prognostics and Health Management (PHM) and Reliability-Centered Maintenance (RCM), creating an industry-leading monitoring solution for rotating equipment.
Integration of PHM (Predictive Health Management) in the AI Model
The core of PHM lies in predicting and managing equipment health. The concept is reflected in the following areas:
1. Health Status Evaluation and Trend Prediction:The AI model uses multi-feature data and trend analysis to predict the equipment’s health status. The trend graph (e.g., color bars) clearly shows the probability of each state, helping to quickly assess health trends. By standardizing the modeling approach, health status models are built for different equipment types to ensure comparability of results. The expansion of data sources (e.g., temperature, pressure, and environmental conditions) improves the accuracy of health status evaluations.
2. Anomaly Detection and Early Warning:The AI model can detect subtle but critical feature changes, providing early warnings for potential failures and avoiding intervention only after complete failure. For example, while ISO vibration standards are strict, AI can further incorporate other data points to detect anomalies that traditional methods miss. The model generates personalized early warnings for each piece of equipment based on historical data, with multi-tiered warning strategies to distinguish between minor and serious anomalies. This helps avoid false alarms and ensures smooth operations. Standard operating procedures (SOPs) for anomaly response, including inspections, diagnostics, and scheduling, are provided.
3. Dynamic Optimization and Learning:PHM emphasizes data accumulation and dynamic model updates. The AI model continues to learn new data based on real-world applications and gradually optimizes prediction accuracy. The platform supports continuous learning, automatically using new data to train and update the model. It allows collaboration with equipment suppliers or users for model validation and optimization.
4. Decision Support:The AI model provides maintenance recommendations based on the health status, such as suggesting scheduled maintenance when the state is in “warning,” rather than immediate shutdown, maximizing equipment usage efficiency and providing decision-making reference for management.
Integration of RCM (Reliability-Centered Maintenance) in the AI Model
RCM focuses on systematic analysis to choose appropriate maintenance strategies that improve equipment reliability. The concept is reflected in the following areas:
1. Function-Oriented Analysis:The AI model monitors rotating equipment based on functional requirements, ensuring mechanical operations meet usage goals (e.g., stable vibration data). It focuses on the health of core equipment functions rather than surface issues of individual components. Key performance indicators (KPIs) are defined for each equipment, such as vibration speed and acceleration, while adapting to different industrial scenarios.
2. Failure Mode and Analysis:The AI model builds failure mode models using characteristic data, identifying the key impacts of failures on production. For example, when vibration anomalies are detected in the Y-direction, AI combines other features for a full analysis, avoiding partial judgments. A shared failure database is created to focus on predicting and analyzing common failure modes.
3. Optimizing Maintenance Strategies:RCM advocates choosing the most appropriate maintenance method (e.g., preventive maintenance, condition-based maintenance, or corrective maintenance). The AI model dynamically determines whether immediate repairs are needed or if regular checks should be scheduled based on health status and trends. Maintenance strategies are established based on health status levels, and the impact of different maintenance modes on production is simulated to find the optimal solution. Maintenance plans are automatically updated based on real-time monitoring data.
4. Cost-Benefit Balance:Through AI’s accurate predictions, excessive maintenance is avoided (lowering maintenance costs), and equipment failures due to lack of monitoring are prevented (reducing production loss), thus lowering overall equipment maintenance costs. Additionally, resource allocation is optimized based on health status and maintenance needs, dynamically distributing repair personnel and parts. Health status monitoring models tailored for different industrial fields (e.g., semiconductors, petrochemicals, and power generation) ensure the best adaptability.
Battle Situation Platform and Monitoring System
Exclusive Dashboard for Small-Scale Operations Room Integration
Rotating Machinery Equipment Monitoring IoT
Comprehensive Monitoring for All Rotating Machinery in the Factory
Portable Inspection Measurement Instruments
Plug-and-Play, Ideal for On-Site Inspection Personnel
Yield Improvement through Data Management
Planning Suitable Measurement Solutions for Different Fields, Providing a Basis for Improving Production Line Management Quality and Predictive Maintenance of Equipment.