Manufacturing Equipment Monitoring
Technology Concept|Manufacturing Equipment Monitoring
Various manufacturing equipment can be monitored using machine learning.
Good Tech's VMS-ML machine learning monitoring system is applicable to any machine with repetitive motion patterns,
enabling real-time detection of dynamic mechanical motion signals.
Even minor anomalies can be detected in advance, allowing early identification of potential machine failures.
Reasons for Implementation
Why use machine learning for equipment monitoring?
In the era of smart manufacturing, factories are filled with automated production machinery. These machines, composed of various mechanical structures and electrical systems, perform different production tasks and undergo repetitive cyclic processing.
Examples include machining centers, robotic arms, CNC machines, stamping and forging presses,
automated welding robots, and injection molding machines.
The operational behavior of such machines is highly complex, and
normal and abnormal signals often overlap, making simple numerical thresholds insufficient for detecting anomalies.
Therefore, a single numerical threshold for warnings is no longer adequate.
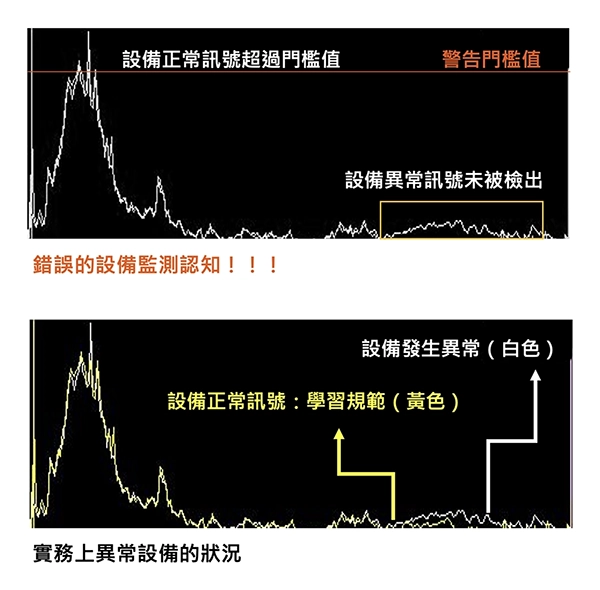
Core Concept
Providing More Effective and Faster Solutions!
・Automation of Equipment ・ Unmanned Operations (Human-Machine Ratio) ・ Smart Equipment
Big Data
Machine-Learning
AI
Avoid Human Errors & Optimize Analysis Process
Convert Analytical Knowledge into Product Functions
Simplify Monitoring Categories & Achieve Precise Classification
Achieve True Expandability
Optimize Decision-Making and Provide More Effective, Faster Solutions
How to Execute
1. Learn the Correct Standards for Comparison
Utilizing the characteristics of machine learning,
the system quickly establishes the correct learning standards for equipment,
compares them with real-time dynamic signals, and detects anomalies instantly,
eliminating the need for long-term data collection.
The system is already categorized by machining behavior and process types, allowing
clean process databases to be directly incorporated into event execution training.
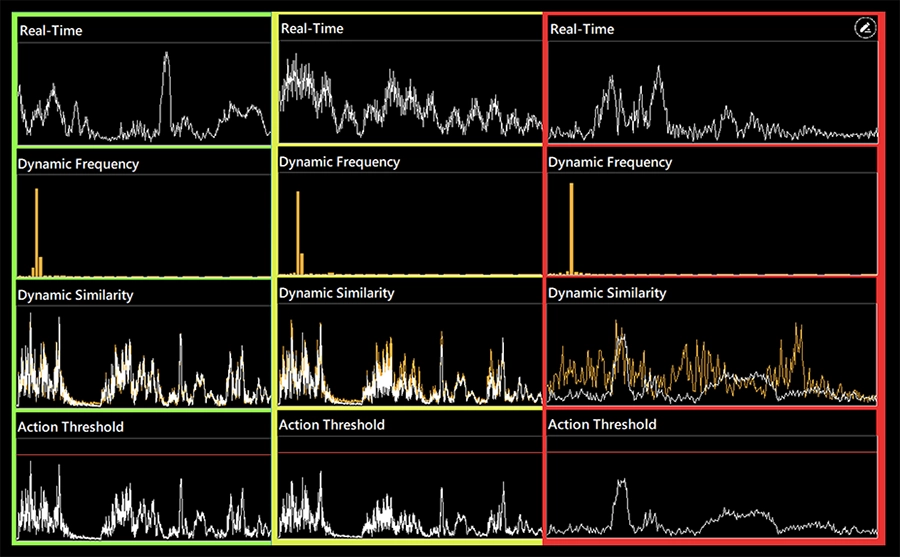
Establishing Learning Standards
The system learns the correct dynamic signals of the equipment and selects the key signal intervals for the process. Multiple dynamic signals can be stored, and users can manually label different mechanical actions to create separate learning standards.
Extracting Necessary Dynamic Signal Intervals
By integrating user experience, selecting only the necessary dynamic signals avoids unnecessary data accumulation
and enhances software processing efficiency.
Users can label specific actions to quickly identify when process or motion abnormalities occur.
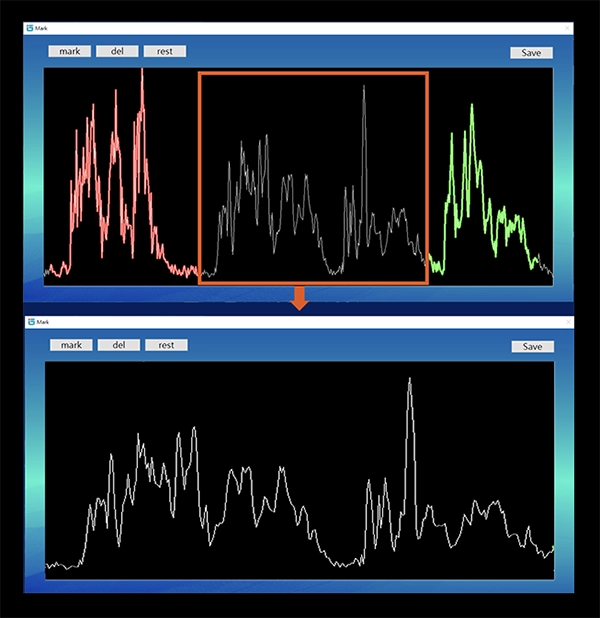
How to Execute
2. Automatic Tracking and Identification Technology
The system learns and records different motion models of the machine.
When the machine performs different actions, the system automatically matches the real-time signal with its model library
to determine if the signals are identical, using this model for monitoring.
Even if anomalies occur in the manufacturing process or external interference is present,
the automatic tracking and identification technology can still accurately determine whether the data signals belong
to the same cycle of motion.
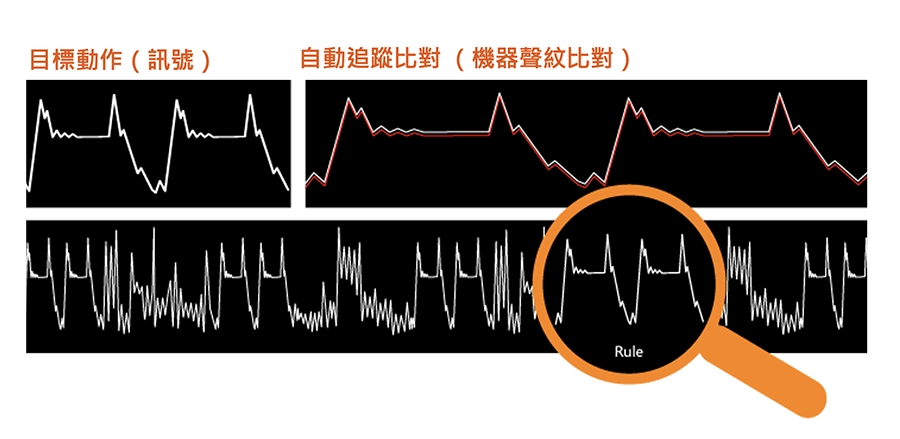
Machine Acoustic Fingerprint Matching
Machine acoustic fingerprint matching is a technology that analyzes and compares unique sound wave characteristics
to identify or distinguish a specific machine.
Just like human fingerprints, every machine has unique vibration patterns, frequency characteristics, tonal variations,
and operational rhythms.
Automatic tracking and identification technology extracts key dynamic features such as frequency characteristics,
vibration patterns, and dynamic changes from the signal data.
These features are then used for pattern matching algorithms to determine whether the detected signal is the intended one.
The system incorporates monitoring models including amplitude, frequency, phase, wavelet analysis, standard deviation,
and stability algorithms.
Tracking Even the Most Complex Dynamic Signals
The system quickly learns and calibrates repetitive or partially repetitive production behaviors,
converting human experience into a rule-based monitoring system.
It features high-speed sampling and signal comparison capabilities, allowing analysis of dynamic signals
within approximately 0.2 seconds per cycle, ensuring even the smallest signals are captured.
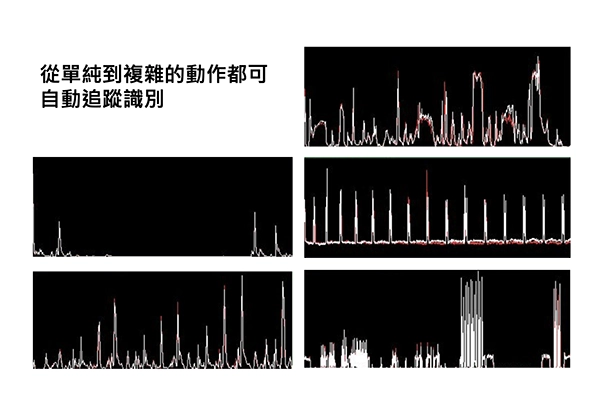
How to Execute
3. Result Scoring Mechanism
The system simplifies complex and vast amounts of data into a scoring mechanism, making it easier for users to interpret.
Outer layer - Machine Health Score: Combines real-time dynamic machine conditions such as similarity score,
vibration exceedance count, frequency exceedance count, machine operation status, and an overall score.
Inner layer - Dynamic Operation Score: Green light indicates normal, yellow light signals a warning, and red light denotes an anomaly.
Real-time vibration signals are automatically compared with predefined standards, and when a match is found,
the corresponding evaluation result is displayed.
Scoring and Weighting
The base weight distribution is derived from large-scale feature value data collection and the accumulation of
machine performance data (both good and faulty).
Using an AI training engine, key feature values are extracted based on machine type characteristics.
These values are then weighted optimally according to their importance in machine operation,
resulting in a normalized scoring distribution.
Indicator Light Display Explanation
・If monitoring status is excellent, the indicator light displays green (Pass).
・If the score is within the Health / Health Normal range, the indicator turns yellow.
・If the score falls below the threshold, it is classified as an anomaly, and the indicator shows red (Fail).
・Mechanical State: If the machine state bar's Pass ratio is
above the threshold, it is green,
below the threshold, it is red.
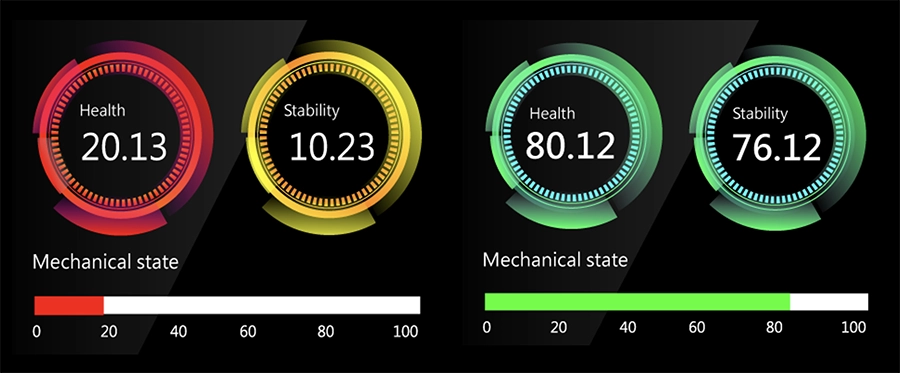
How to Execute
4. AI-Based Feature Accumulation
The system collects a vast amount of raw data, some of which may be unnecessary.
By cleaning and refining the data, system efficiency is improved, and data features are standardized,
allowing various types of data to be displayed uniformly in the UI.
Additionally, by accumulating feature values, the system generates trend graphs,
making **secondary threshold management** easier for users.
The feature trend graph visualizes the aging process of machines, enabling users to define
future maintenance plans based on trend data.
With AI training, the system can also predict potential variations that may occur in the future.
AI-Based System for Machine Feature Recognition
Traditional AI-based monitoring systems require extensive data accumulation and a significant number of recorded events,
making the initial cost of building a model high and its effectiveness difficult to estimate.
Even if a large amount of data is collected, it cannot be effectively utilized without proper data cleaning and feature extraction.
Furthermore, each type of data requires collaboration between domain experts and data scientists to be properly analyzed and modeled.
By the time an effective system is implemented, a substantial amount of money and time has already been spent.
Machine Learning Intelligent Monitoring System
VMS-ML eliminates the long process of data collection and delivers immediate benefits.
By selecting sensors directly relevant to the characteristics of the manufacturing process and monitoring items,
it ensures more accurate data collection for analysis and monitoring.
With a non-intrusive measurement approach, there is no need for complex equipment integration,
and the system starts delivering value from the moment of installation, instantly performing production line monitoring.
Machine Learning Intelligent Monitoring System Application Cases
Application to Various Machinery
The VMS®-ML Machine Learning Intelligent Monitoring System can be applied to any machinery with repetitive motion.
It enables real-time detection of dynamic machine movement signals.
Even minor anomalies can be detected in advance, allowing users to determine if a machine is developing signs of failure.
This provides ample time to prepare for component replacement or production line scheduling, **preventing unexpected breakdowns that could impact operations and lead to losses.**
Additionally, it effectively prevents quality issues caused by machines operating in abnormal conditions.
Application Scope:
・Any machinery with repetitive motion behavior
・Parallel robotic arms for wafer handling
・Multi-axis robotic arms
・Pick & Place devices
・Automated production line equipment
・Semiconductor lithography equipment, cleaning machines, parametric coating equipment
・Packaging plants (front-end): Dicing machines, wafer loaders, wire bonders, laser cutters, grinding machines
・Packaging plants (back-end): Header, probe stations
・Large transport robotic arms in display manufacturing plants
・Rotary coaters and parallel coaters in display manufacturing plants
Support
Other Machine Learning Applications