Predictive Maintenance for Cyclical Production Equipment
Solutions|Predictive Maintenance for Cyclical Production EquipmentIn the era of smart manufacturing, factories are filled with numerous automated production machines. These machines, composed of various mechanisms and motors, perform different production tasks while carrying out repetitive cyclical processing, classifying them as cyclical (repetitive) production equipment.
Why Implement?
Enhancing Equipment Productivity and Production Line Yield
Implementing Accurate Predictive Maintenance
In recent years, industrial manufacturing has placed significant emphasis on improving product yield and production capacity. As manufacturing processes become more precise, the operational demands on equipment also increase. By conducting real-time equipment monitoring and collecting large-scale data, we can develop predictive maintenance plans. Identifying early signs of equipment failure helps prevent unexpected downtime, maintain seamless production, ensure product quality, protect equipment integrity, optimize manufacturing processes, reduce costs, and ultimately improve both production line yield and capacity.
What is Cyclical (Repetitive) Production Equipment?
In smart manufacturing, factories are filled with automated production machines. These machines, composed of various mechanisms and motors, perform different production tasks while carrying out repetitive cyclical processing, classifying them as cyclical (repetitive) production equipment. Examples include machining centers, robotic arms, CNC tools, press forging machines, automated welding robots, and injection molding machines.
Achieving Zero Defects in Production
PHM and PDM primarily focus on predictive maintenance. In industrial production, product quality is the most critical factor. The most proactive and effective solution is to integrate production quality control. AOI (Automated Optical Inspection) combined with AI has become a standard quality control method to prevent defective products from being shipped. However, production line monitoring should take a step further and focus on preventing defects from being created in the first place.
By integrating real-time production monitoring with post-production quality control, we can achieve zero-defect manufacturing as a common goal.
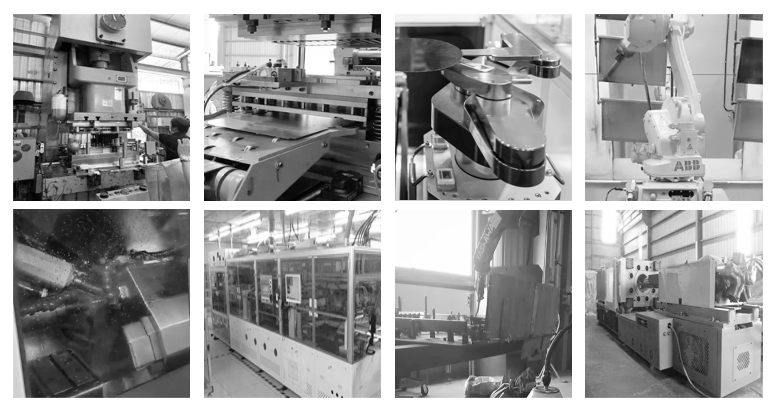
Application Areas
Cyclical Production Equipment
Semiconductor Crystal Growth:
- Ingot Cutting
- Lapping
- Chamfering Machine
- Argon Anneal Robot
- LPCVD Robotic Arm
- Loader Quality Monitoring
- Wet Etching Dryer
- Wafer Polishing Machine
Semiconductor Packaging:
- Dicing Machine
- Grinding Machine
- Wafer Transfer Machine
- Wire Bonder
- Laser Cutting Machine
Semiconductor Manufacturing:
- PVD/CVD Robot
- Wet Transfer Robot
- LPCVD Robotic Arm
- APCVD Robotic Arm
- Transfer Robot
- STK (Stocker)
Mechanical Industry Applications:
- Press Machine
- Servo Press Machine
- Digital Punching Machine
- Punching Machine
- Smartphone Case Milling
- Metal Bending Machine
- Swiss-Type Lathe
- Thread Rolling Machine
Automobile Manufacturing:
- Press Line
- Automated Welding Line
- Large Robotic Arm
Display Panel Manufacturing:
- Panel Cutting Machine
- Coating Process
- Glass Cleaning Process
- WET/Stripper Robot
- PVD / CVD Robot
- Load-to-Load Robot
- Stocker
PCB Industry:
- Cutting Machine
- Drilling Machine
- Edge Grinding Machine
- Target Drilling Machine
Monitoring Essentials
Concept of Equipment Signal Monitoring and Threshold Standards
Using Different Learning Standards Based on Manufacturing Modes
Since the mechanical operations of periodic equipment are highly complex, normal and abnormal signals often overlap during operation. Simply using numerical high or low values is no longer sufficient for determining abnormalities. Therefore, relying solely on a single numerical warning threshold is inadequate.
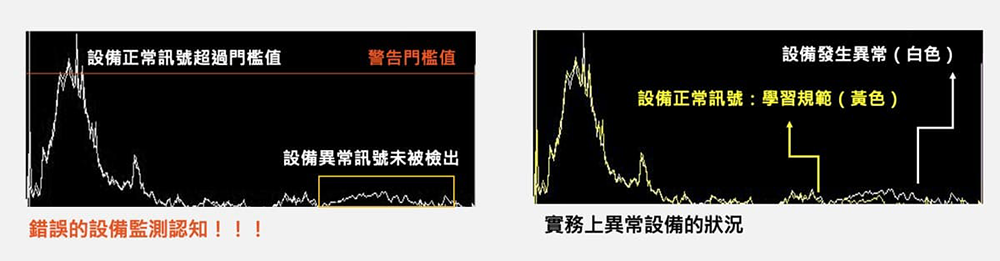
Additionally, for periodic equipment, even the same machine will exhibit different signal patterns depending on the type of product, material, and rotational speed. Therefore, it is necessary to apply different learning standards tailored to each manufacturing mode.
Monitoring Essentials
Combining Relevant Physical Quantity Sensors
Using Different Sensors Based on Process Characteristics
Depending on the characteristics of each manufacturing process, different types of sensors are used. For example, mechanical arms, press forging molds, and machine tools related to mechanical behavior use vibration sensors (accelerometers). Automatic welding, which is directly related to electrical current, uses current sensors, while automated dispensing, coating, and injection molding, which are directly related to pressure, use pressure sensors.
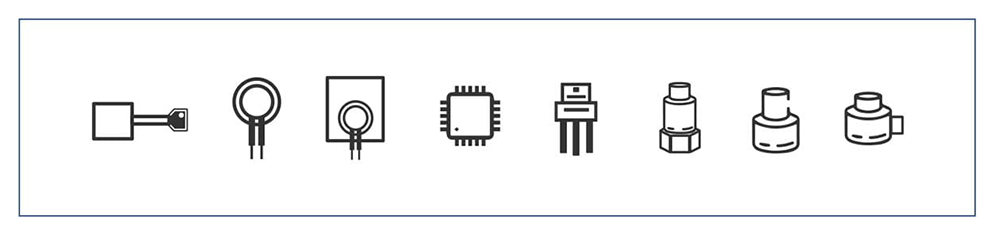
Monitoring Essentials
Eliminating the Long Data Collection Process
A System That Generates Immediate Benefits Upon Installation to Execute Production Line Monitoring
Traditional AI monitoring systems require long-term accumulation of vast amounts of data and numerous case studies before constructing a model, making it difficult to estimate the cost-effectiveness upfront. Even if a large amount of data is collected, it cannot be effectively utilized without proper data cleaning or feature extraction. Furthermore, each type of data requires collaboration between domain experts and data scientists to reanalyze and model, leading to excessive time and financial costs before any actual benefits can be realized. To solve this issue, a system that provides immediate benefits upon installation and can start executing production line monitoring tasks right away is essential.
Pain Point Solution
Difficulty in Integrating Monitoring Systems with Equipment Data?
Periodic equipment has complex movements and mechanisms. How should measurement points be installed? Since machine operations are diverse and complicated, integrating data between machines is challenging. Furthermore, different machines are manufactured by different companies, making data acquisition and integration even more difficult. How can this be overcome?
Solution
Non-intrusive measurement eliminates machine data integration
The VMS-ML Machine Learning Smart Monitoring System eliminates the need for machine data integration by adopting a non-intrusive measurement approach. With true PLUG-IN functionality, a single sensor based on key process monitoring indicators can be installed, allowing immediate execution of production line quality monitoring tasks.
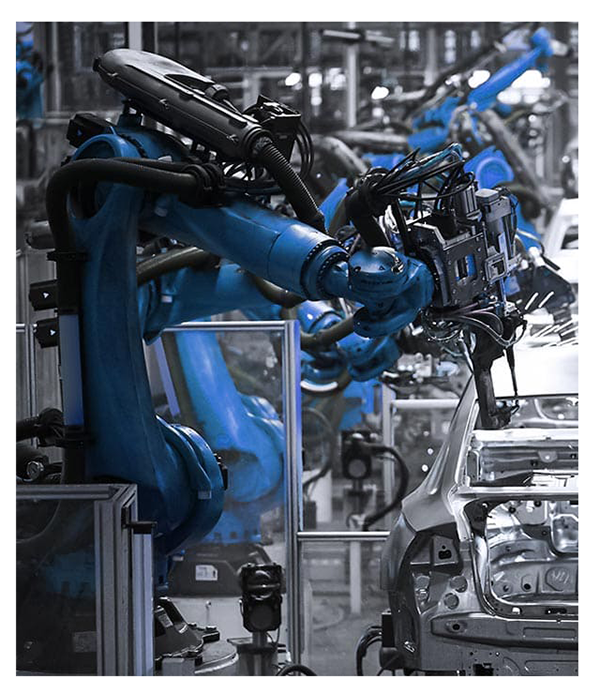
Pain Point Solution
Complex factory environments make signal interpretation difficult?
Automated factories are filled with numerous automated machines operating simultaneously during the manufacturing process. Additionally, uncontrollable factors such as earthquakes or external construction can cause significant interference. How can we correctly interpret the collected data in such environments?
Solution
Automatically track target signals and determine results
VMS-ML automatically tracks target processing signals. During real-time monitoring, it detects predefined target signals automatically. Even when mechanical motion anomalies or external interference occur, the system’s automatic tracking technology can accurately determine whether the data signal belongs to the same set of periodic operations and provides a judgment result.
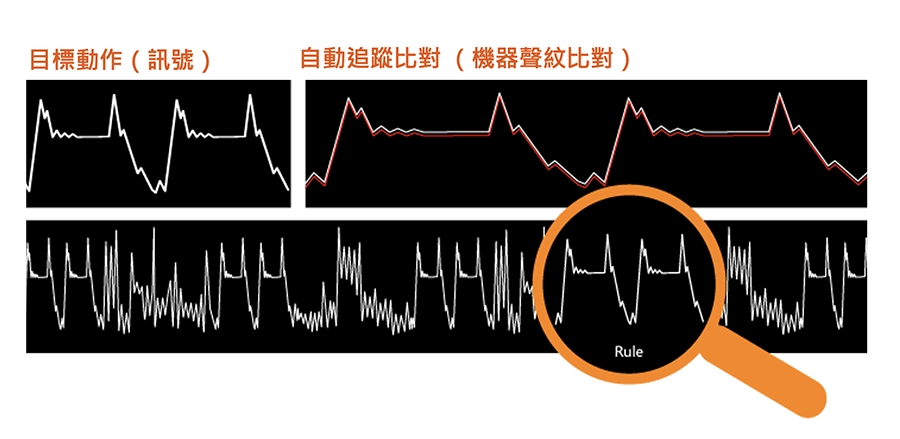
Pain Point Solution
Diverse equipment types make multiple monitoring methods burdensome?
Periodic production equipment varies widely in type and complexity, and different machines require different monitoring methods. As a result, maintenance engineers must familiarize themselves with multiple monitoring systems. In some cases, different engineers are responsible for different machines, making operations and maintenance inefficient. Is there a single solution that can meet the monitoring needs of all periodic production equipment?
Solution
One system for multiple equipment monitoring
VMS-ML is a monitoring system specifically designed for periodic production equipment. It supports a multi-channel version, allowing a single system to monitor multiple machines.
The system uses algorithms to display key results in the simplest form of red-yellow-green signal lights and scores.
It retains detailed data scores and feature maps, offering multiple predefined monitoring criteria for users to customize. With a user-friendly interface, engineers can start monitoring immediately after brief training.
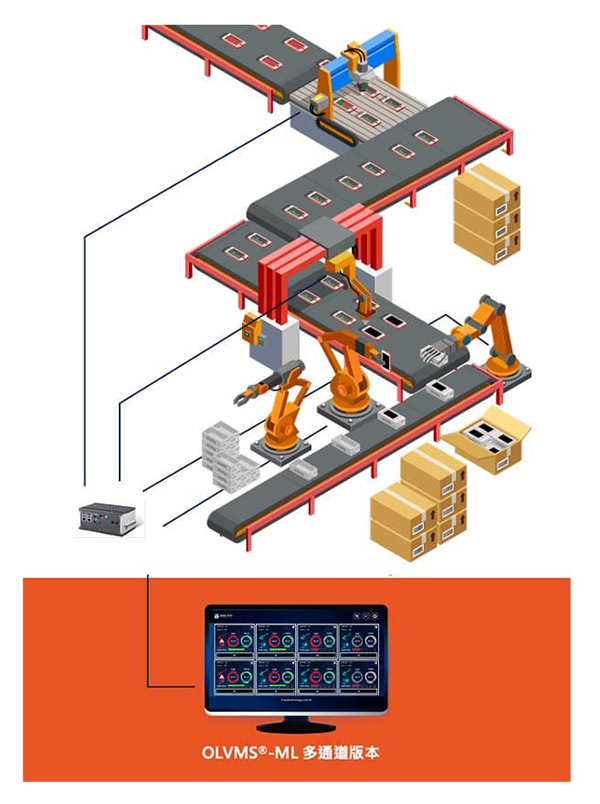
Pain Point Solution
AI requires a long data collection period, delaying benefits?
AI-based monitoring systems often require extensive data collection and accumulation before they can extract meaningful features from abnormal events.
When encountering different types of incidents or processes, additional data accumulation is required.
As a result, the time until the monitoring system can perform tasks remains uncertain. The long data collection period delays realizing the benefits of monitoring. How can we solve this?
Solution
Rapidly establish monitoring criteria and detect anomalies instantly
VMS-ML allows rapid setup of learning criteria, enabling instant detection of anomalies in equipment without prolonged data collection periods.
The system already categorizes clean manufacturing process data, allowing immediate training execution.
Additionally, built-in monitoring models utilize algorithms such as amplitude, frequency, phase, wavelet analysis, standard deviation, and stability to quickly analyze signals and generate results.
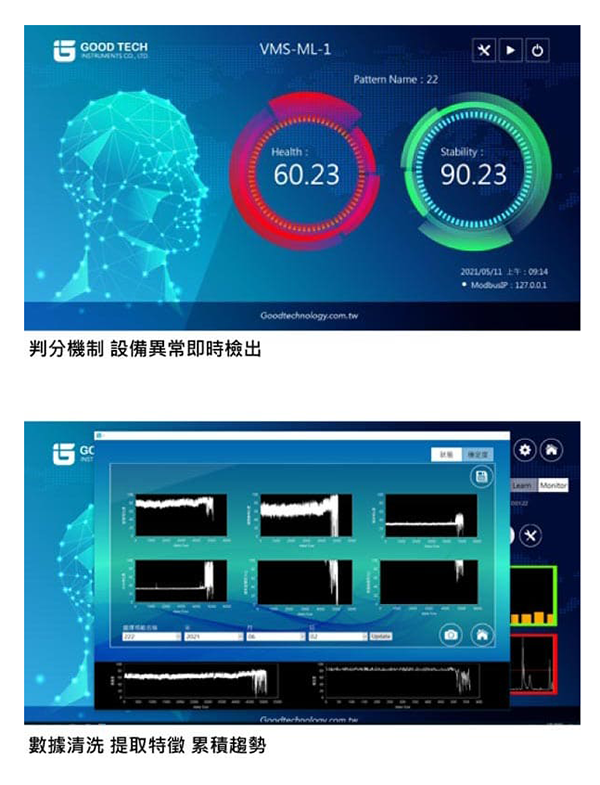
Pain Point Solution
How to unify inspection standards through data?
In the manufacturing and machining process, variability in human inspection and experience-based judgment often leads to inconsistencies in quality standards.
Minor defects may go undetected, resulting in low inspection efficiency. How can we unify standards to prevent inconsistent quality?
Implementation
Establishing a Unified Data-Based Inspection Model
VMS-ML rapidly learns (calibrates) repetitive or partially repetitive production behaviors and converts human expertise into a rule-based monitoring system.
By defining production cycle regulations based on manufacturing experience, the system effectively establishes a unified data-based inspection model, ensuring standardized product quality.
・Define damaged components based on equipment timing sequences.
・Users can design custom health check modes.
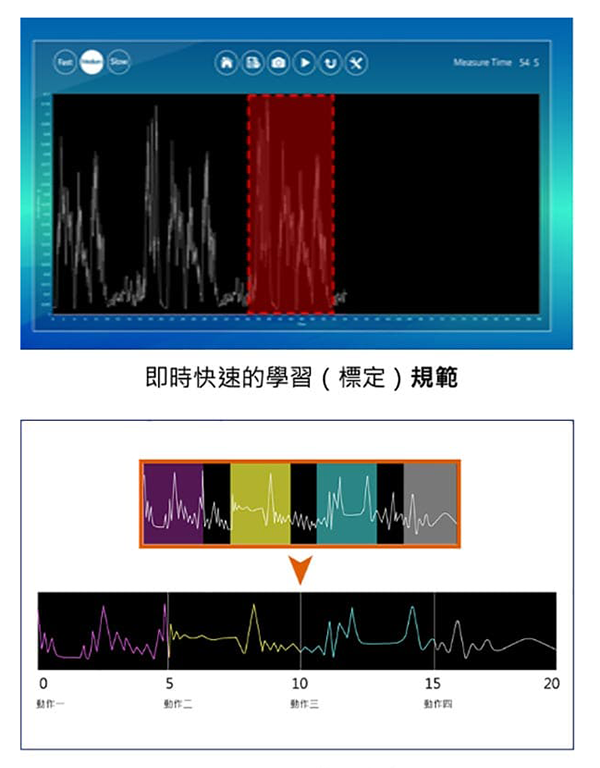
Pain Point Solution
How to eliminate unnecessary data parameter comparisons?
Manufacturing processes vary in duration and complexity. Equipment parameters change based on different products, production actions, or manual operations such as mold changes or movement.
If the system compares data across different actions, the results may become meaningless. Is there a way to filter out unnecessary data?
Implementation
Selectable Learning Intervals
VMS-ML allows users to quickly establish learning criteria by recognizing correct sequences and eliminating irrelevant actions.
The system’s exclusion function enables users to specify actions to be ignored, ensuring that only relevant sequences are analyzed.
Additionally, unnecessary sequences are excluded from storage, optimizing memory usage.
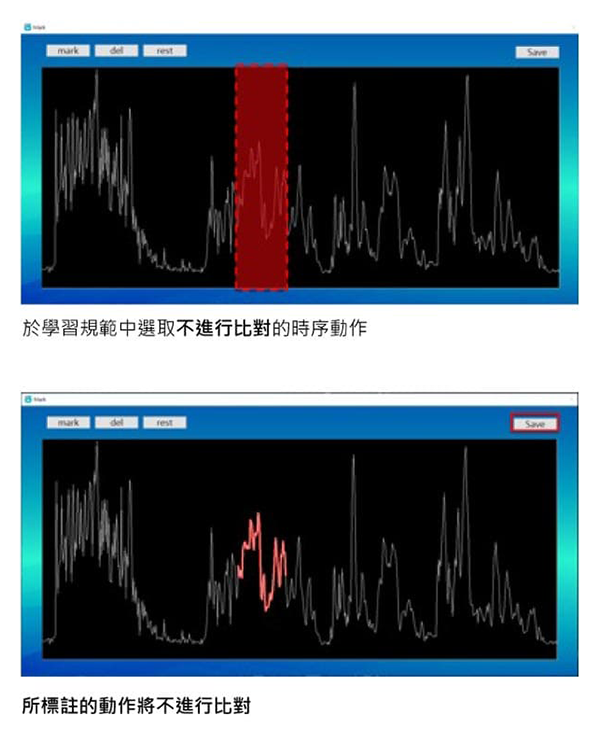
Pain Point Solution
How to prevent large-scale defective production proactively?
High-speed automated production lines may produce massive defects due to minor malfunctions such as excessive vibration causing displacement or accuracy deviation. How can we detect and prevent such issues in advance?
Implementation
Early Detection of Equipment Issues and Future Condition Monitoring
When minor abnormalities occur in the equipment, VMS-ML can instantly detect them.
By utilizing VMS-PH, equipment anomalies can be quickly diagnosed, reducing downtime.
Through predictive maintenance, major failures can be prevented, avoiding the mass production of defective products.
Machine learning behavior models assist operators in establishing standardized processes.
By tagging anomaly characteristics, users can trace back the process, optimize production workflows, and better predict future equipment conditions.
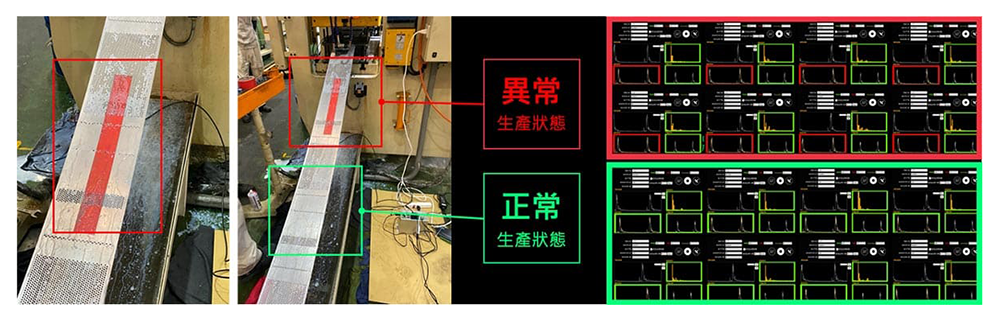
How to Implement
Non-intrusive Measurement, No Machine Integration Required
The VMS-ML Machine Learning Smart Monitoring System does not require machine integration and adopts a non-intrusive measurement method.
It achieves true PLUG-IN functionality—simply install a single sensor relevant to the key manufacturing monitoring indicators, and the system can immediately begin production line quality monitoring tasks.
Application Performance
How to Prevent Wafer Scratches During Transportation?
Using VMS-ML to monitor the robotic arm’s status, preventing wafer damage and scrapping due to abnormal arm behavior.
The system monitors the wafer transport process, issuing alerts for abnormal scratches or collisions.
Simulating abnormal conditions by lightly tapping the fork, changes in dynamic signals and frequency domain
cause a decrease in similarity scoring.
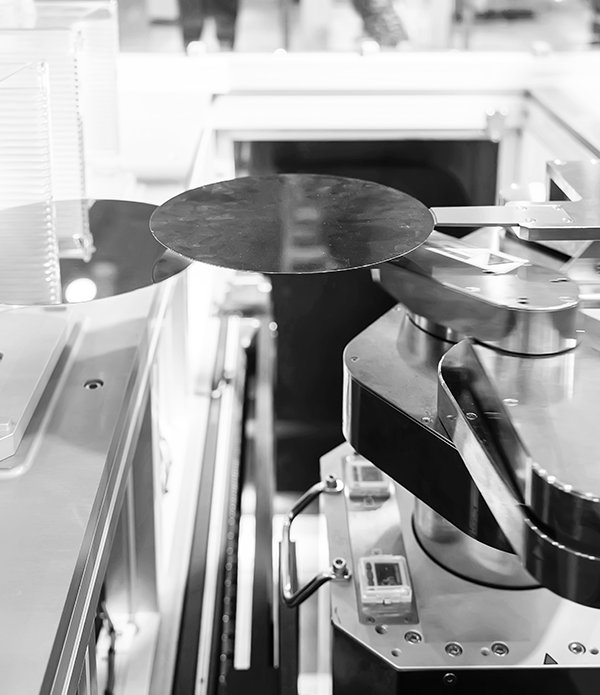
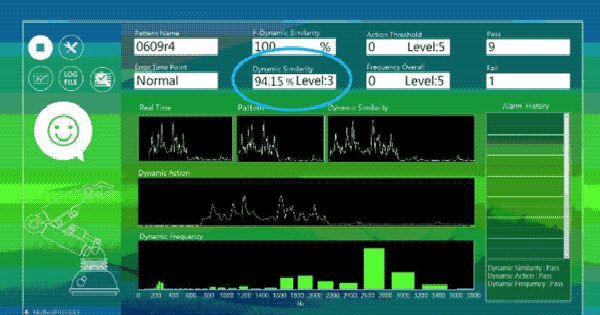
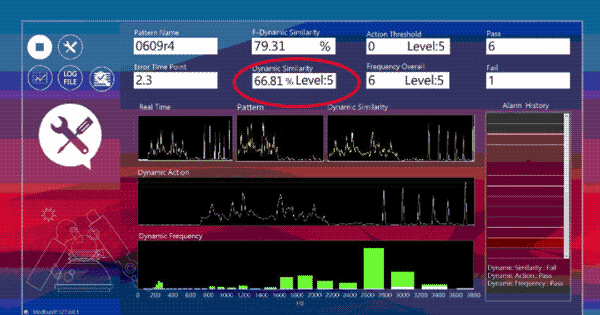
Application Performance
Press Mold Abnormalities Causing Mass Defects?
High-speed stamping machines produce large quantities of products in a short time,
but if mold wear or abnormalities occur, a large number of defective products may be produced.
To ensure efficient and stable production and allow for timely repair or replacement when needed,
a monitoring system capable of predicting mold conditions in advance is essential.
This helps facilitate early mold replacement or adjustments, reducing the risk of damage
and preventing a massive production of defective products.
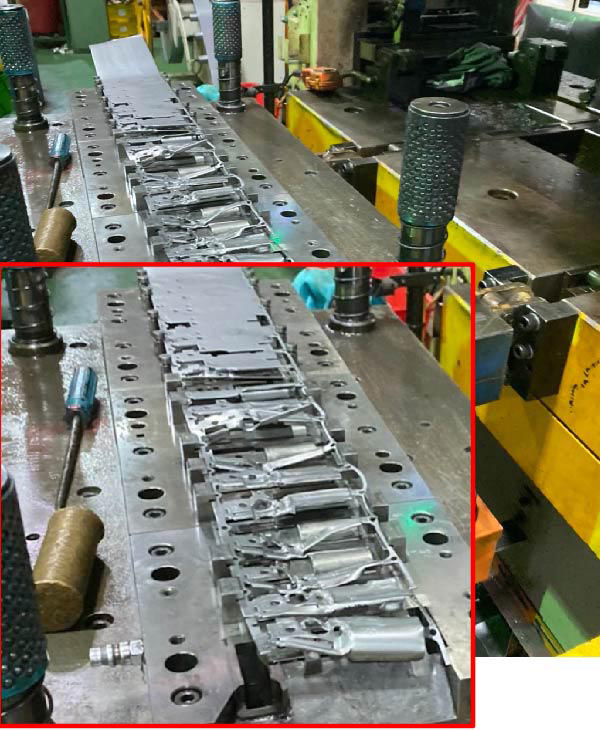
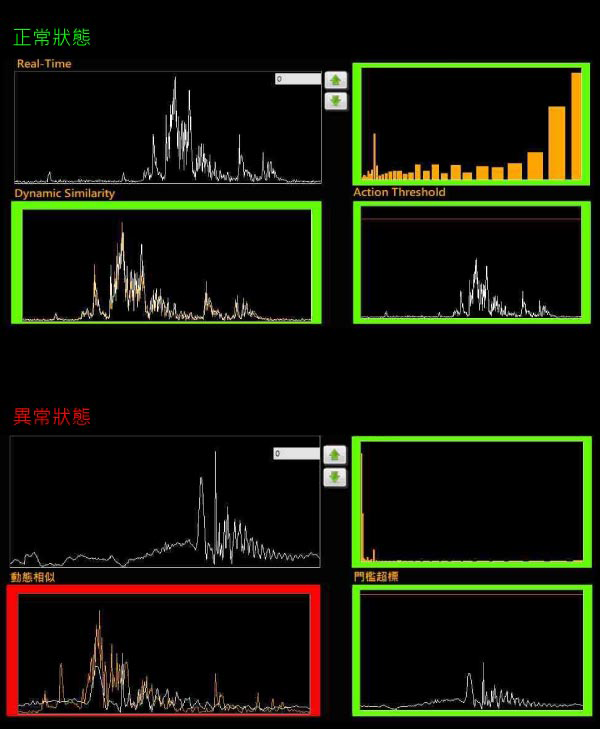
Process Optimization
Periodic Production Equipment PHM
Signal Feature Analysis
As a preprocessing step for periodic production equipment monitoring, VMS®-PH performs
preliminary measurements and analysis of the manufacturing process.
This accelerates ML monitoring effectiveness and enhances AI training.
(If the equipment’s dynamic characteristics are unclear, this step can be skipped.)
Establish Learning Criteria for Monitoring
VMS®-ML provides dynamic monitoring for periodic production equipment,
rapidly establishing learning criteria for monitoring.
The system’s automatic scoring mechanism ensures real-time equipment status awareness.
Establish a Dedicated Control Room
The ML-EDGE IIoT distributed core computing system enables simultaneous monitoring of multiple
periodic production devices while ensuring centralized management through IIoT.
This allows businesses to establish a dedicated control room for equipment supervision.
Quickly and Accurately Identify Anomalies
When the OLVMS®-ML monitoring system detects an anomaly alert,
VMS®-PH helps users quickly and accurately pinpoint the cause of the issue.
This significantly reduces repair time and machine downtime.