Abnormal Stud Welding Causes Poor Object Bonding?
Case|Abnormal Stud Welding Causes Poor Object Bonding?If the stud welding is insufficient or defective, such as porosity, cracks, or cold welding, the connection strength may be compromised.
Stud Welding
Stud welding (also known as through-stud welding or fusion welding) is a metal processing technique commonly used to join two or more metal parts together. The basic principle of this technique is to insert a metal stud (usually an anchor stud) into one or more metal parts and then use high temperature (typically flame or electric arc) to melt the metal, forming a firm connection with the stud.
Stud welding is often used in applications requiring high strength and durability, such as steel structures in buildings, ships, bridges, and pipelines. This technique can be applied to various types of metals, including steel, stainless steel, and aluminum.
How can poor stud welding affect bonding?
Reduced strength: If the stud welding is insufficient or contains defects such as porosity, cracks, or cold welding, the connection strength may be compromised. This can result in breakage or deformation under stress, jeopardizing the stability and durability of the structure.
Leakage: In applications requiring sealing, poor stud welding may lead to leakage, which is particularly critical for applications such as pipelines, containers, and pressure vessels that contain hazardous substances.
Corrosion damage: Poor stud welding may create cracks or holes, making metal surfaces more vulnerable to corrosion, leading to premature degradation and failure of metal components.
Cracks and fractures: Poor welding may cause cracks that can expand over time, leading to connection failure. This poses a threat to the reliability and safety of structural or mechanical components.
Difficult repairs: If the stud welding is defective, repairs or replacements may be required, increasing costs and downtime.
Issues to Monitor:
1. If the welding is insufficient or defective, the connection strength may be compromised.
This can lead to breakage or deformation under stress, threatening the stability and durability of the structure.
2. Welding quality is manually inspected, which often results in inconsistent checks that affect subsequent processes. Automation and data-driven solutions are needed.
How to Detect?
VMS-ML Machine Learning Intelligent Monitoring System
The machine learning intelligent monitoring system utilizes machine learning technology to learn the correct process flow specifications. By integrating process characteristic sensors, even the smallest signals are captured. In this case, current sensors are used to learn and monitor the welding process, helping to determine whether the stud welding quality is acceptable.
Measurement Conditions
Measurement Condition 1
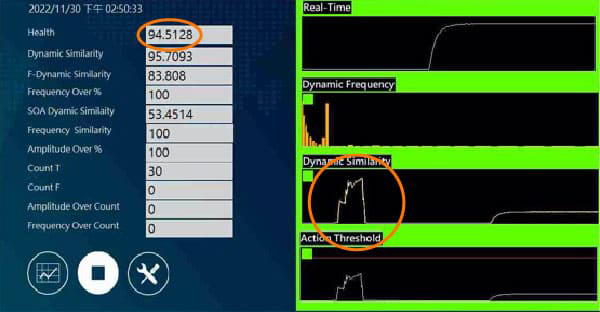
Measurement Condition 1: Normal Stud Welding Voltage
Result: Successfully identified, score: 94.513.
Result: PASS
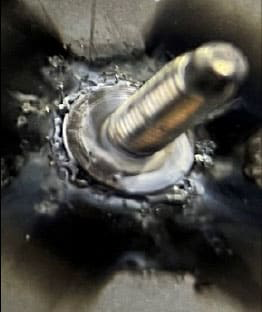
Measurement Condition 2
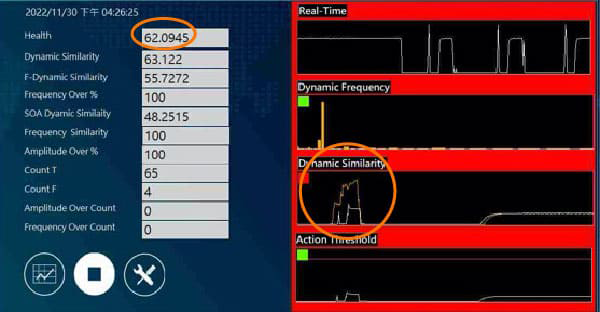
Measurement Condition 2: Abnormal Stud Welding Voltage (Short Circuit Voltage)
Result: Health score 62.095 (below the preset threshold of 80, determined as NG)
Result: FAIL
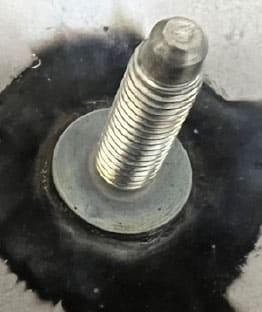
Measurement Conclusion
VMS-ML can promptly intercept defective products. From the above experiment, we can see that when defects occur in the production process, the system can instantly capture abnormal signals and provide alerts. This eliminates the need for manual quality judgment, avoiding human errors.
Additionally, the trend graph allows users to anticipate equipment anomalies early and perform predictive maintenance in real-time. This helps users understand the production line status and take timely actions to improve product quality.