Poor Welding Environment? Inconsistent Manual Inspection Quality?
Case|Poor Welding Environment? Inconsistent Manual Inspection Quality?Welding is a high-temperature, high-pressure, and high-intensity job. The repetitive nature of manual feeding actions, combined with poor working conditions, makes it difficult to recruit workers.
Automated Welding Technology
Automated welding technology is increasingly prevalent in the manufacturing industry and has become an indispensable part of modern production lines. It offers advantages such as improved production efficiency, reduced labor costs, and consistent product quality. However, its implementation also comes with many challenges and bottlenecks. Particularly, the repetitive nature of manual feeding actions and the harsh welding environment make it difficult to recruit operators. Welding involves high temperatures, high pressures, and high workloads, which, over extended periods, can have adverse effects on operators' physical and mental health. The repetitive nature of feeding actions makes it difficult for operators to maintain efficiency and focus, further reducing productivity and production quality. These working conditions make it challenging for companies to attract and retain skilled personnel.
Does manual inspection result in inconsistent quality and affect production efficiency?
Manual inspection methods for welding quality have significant shortcomings. Traditional manual inspection methods rely on the experience and visual judgment of operators, which can be time-consuming and subject to individual bias, leading to inconsistent results. Particularly in large-scale production, the efficiency and accuracy of manual inspection may not meet high production demands, causing fluctuations and instability in welding quality. When welding defects occur, rework is often required, which increases production costs and extends production cycles, negatively impacting overall efficiency.
Solution and Monitoring Description
VMS-ML Machine Learning Intelligent Monitoring System
In addition to the development of fully automated production line workstations, monitoring of production line operation status, equipment dynamics, and wear remains crucial even with reduced human intervention. This project introduces an intelligent monitoring system to manage production processes. Gude Technology utilizes vibration and current sensors to collect external signals from equipment and perform calculations, establishing similarity comparison benchmarks and trend thresholds. This system operates independently without needing direct machine connections and communication, allowing autonomous monitoring and management. In the future, all intelligent monitoring systems across the factory can be integrated into a centralized management system.
The machine learning real-time comparison technology enables monitoring of spot welding operations to distinguish between normal and abnormal behavior. By analyzing operational signals, the system helps assess welding quality, effectively improving product yield rates, standardizing product quality, and reducing operational costs, thus achieving a scientific approach to industrial component manufacturing.
Measurement Status
Normal Motor Rotor Condition
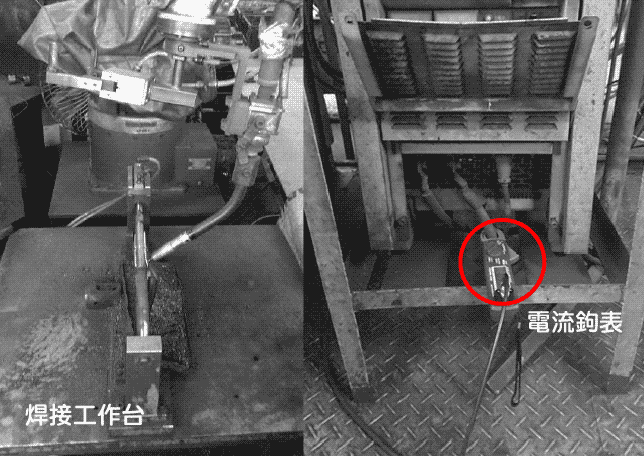
Case Study Verification
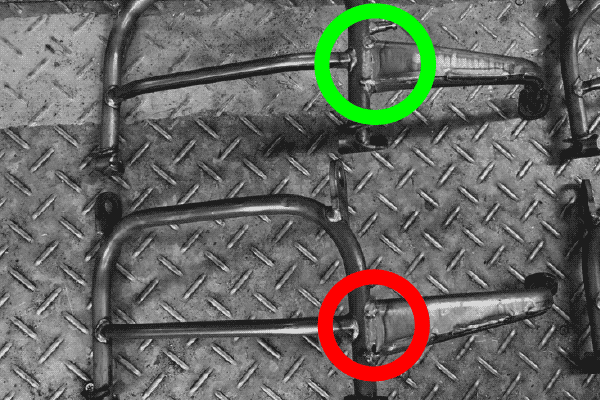
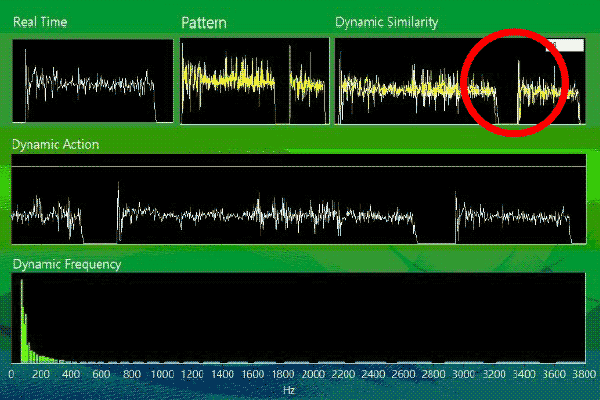
Normal Welding Condition
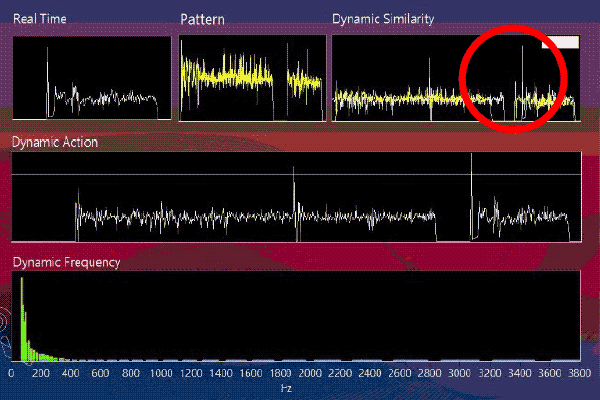
Abnormal Welding Condition
Comparison of Production Line Normal and Welding Leakage Current
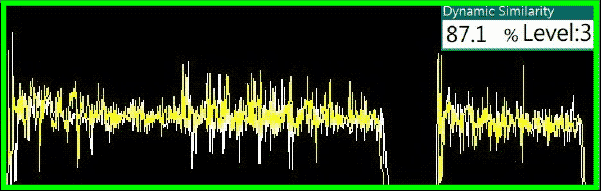
Normal Production
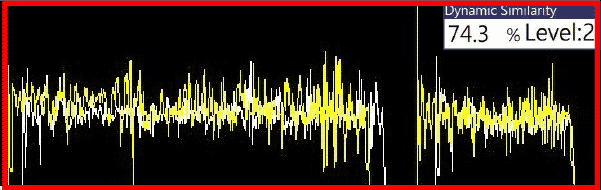
Welding Leakage Current
Measurement Conclusion
To address the above challenges and improve the efficiency and quality of automated welding processes, companies need to take a multi-faceted approach. On one hand, the introduction of advanced automatic feeding systems can reduce manual intervention. Automatic feeding systems, through robotic arms and conveyor belts, achieve full automation of the feeding process, improving efficiency and accuracy while reducing the workload of operators and enhancing the working environment.
On the other hand, implementing machine learning systems with specialized algorithms and current sensors can quickly and accurately detect welding quality defects, significantly improving inspection efficiency and accuracy while eliminating the subjectivity and inconsistency of manual inspection. Enhancing job satisfaction and professional skills among operators further boosts production efficiency and product quality.
In conclusion, the promotion and application of automated welding technology require not only advanced technologies and equipment but also improvements in personnel management and working conditions. Only by addressing these aspects can companies achieve both production efficiency and product quality enhancements, enabling them to stay competitive and achieve sustainable development.
VMS-ML Machine Learning Intelligent Monitoring System