Robotic Arm Smart Monitoring
Solutions | Robotic Arm Smart MonitoringRobotic arms are a crucial component of automation, and their development is expected to continue evolving towards higher efficiency, intelligence, and lighter designs. This helps reduce costs, alleviate labor shortages, accelerate processes, unlock professional expertise, and enhance labor quality, ultimately achieving a smart factory layout.
Reasons for Implementation
Accelerating Smart Factory Deployment
Key Equipment for Smart Manufacturing - Robotic Arms
The global market for robotic arms is rapidly expanding, driven by the widespread adoption of industrial automation, the rise of smart manufacturing, increasing technological demands, cost reduction efforts, and growing demand across various industries. As a core element of automation, robotic arms are expected to continue advancing towards higher efficiency, greater intelligence, and more lightweight designs. This contributes to cost savings, reduces labor shortages, optimizes processes, leverages specialized expertise, and improves working conditions, accelerating the realization of smart factory implementation.
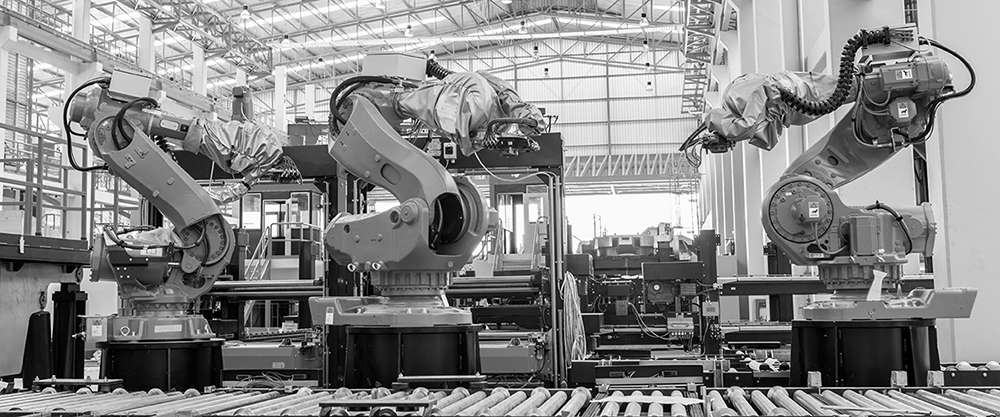
Automatic Welding Robot Arm Monitoring
Reason for Implementation
Welding abnormalities can negatively impact subsequent structures.
There are many factors that affect welding quality. Excessive welding speed may prevent the metal from fully melting and bonding, while welding too slowly can cause overheating and deformation. Incorrect current and voltage settings can result in uneven welding, increasing the risk of defective or weak welds.
These defects affect welding quality and assembly precision, potentially requiring rework or adjustments. In severe cases, they may compromise the strength and durability of welded joints, leading to part failure during use, which can impact vehicle safety and reliability.
Common Issues in the Welding Process:
・Undercut: Incorrect welding torch angle or position.
・Torch Collision: Misalignment in part assembly or incorrect TCP (Tool Center Point) of the welding torch.
・Incomplete Weld: Welding seam is discontinuous.
・Weld Deviation: Incorrect welding position or tracking issues with the welding torch.
・Lack of Fusion: The weld metal does not completely fuse with the base material or between layers.
・Burn-Through: Excessive heat causes holes in the base material.
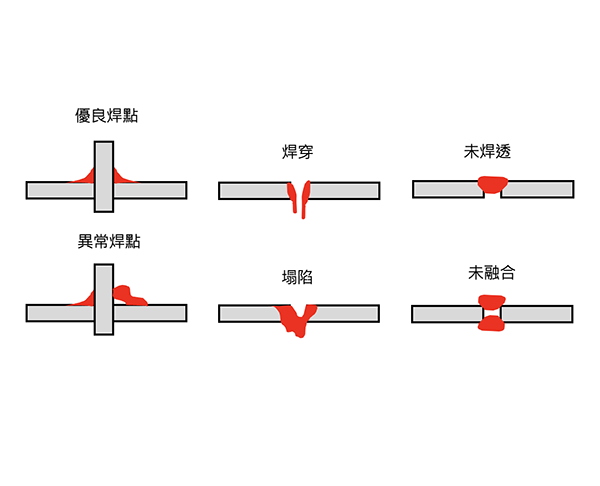
Implementation Challenges
Poor working conditions leading to labor shortages?
Welding work requires not only skilled techniques but also involves repetitive tasks and monotonous manual material handling.
Additionally, the working environment often includes high temperatures, loud noise, smoke, and hazardous gases, making it difficult to recruit and retain workers.
Implementation Method
Introducing automation to reduce labor resources
By utilizing the VMS®-ML Machine Learning Intelligent Monitoring System, automated monitoring is used for process management.
By analyzing equipment operation signals, the welding quality is assessed, and standardized processes and quality control are established, effectively reducing labor resources and factory operating costs.
This enables scientific management in industrial component production.
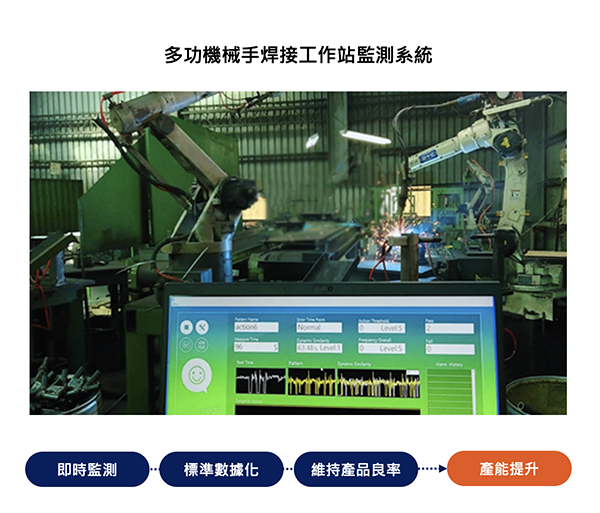
Implementation Challenges
Manual inspection methods result in inconsistent quality?
Manual inspection in mass production environments can significantly reduce overall production efficiency.
Additionally, inspectors performing high-intensity observation and operations for extended periods may experience fatigue and reduced attention, affecting the accuracy of inspection results.
Some welding defects, such as internal cracks or microscopic pores, may not be detectable through visual inspection, potentially compromising the final product's quality and reliability.
Implementation Method
Replacing manual inspection with data analysis to ensure product consistency
By utilizing the VMS®-ML Machine Learning Intelligent Monitoring System, data can be recorded and tracked, effectively reducing the limitations of manual inspection.
This enhances inspection consistency and traceability, ensuring the final product's quality and reliability.
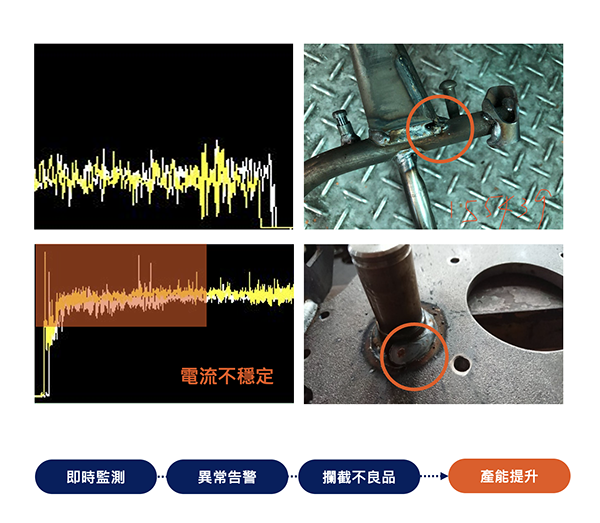
Implementation Challenges
Complex robotic arm movements make monitoring difficult?
Welding robotic arms have diverse movements and complex mechanisms, making sensor deployment and installation challenging.
Additionally, different robotic arms are produced by various manufacturers, and their machine data is managed by third-party equipment providers, leading to difficulties in system integration.
Implementation Method
No system integration required—monitoring starts upon installation
The VMS®-ML Machine Learning Intelligent Monitoring System enables non-intrusive measurement without requiring system integration, making true "PLUG & IN" possible.
Simply install a physical sensor corresponding to key process monitoring parameters (e.g., a current clamp meter for welding robotic arms) and calibrate the current reference points.
This allows real-time monitoring of welding arm current and voltage to manage the most critical process variables in welding operations.
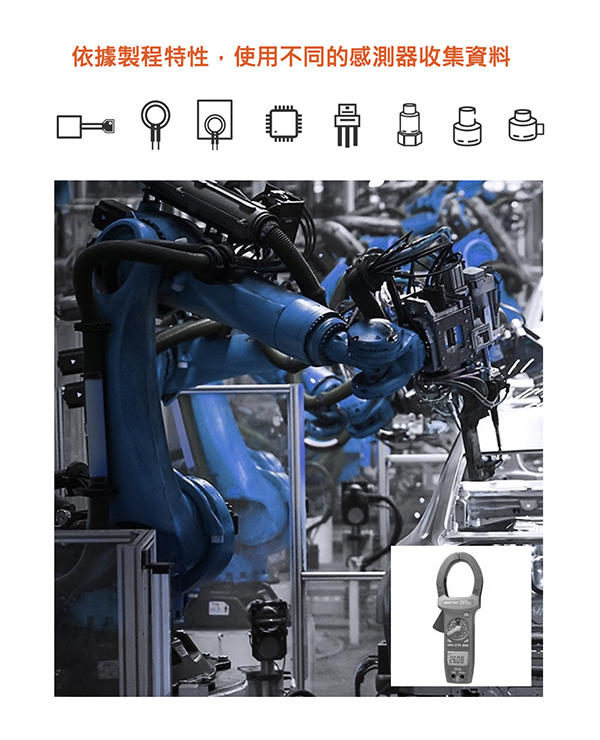
VMS®-ML Machine Learning Intelligent Monitoring System
Designed for dynamic monitoring of various mechanical equipment, users can easily set health criteria.
The system learns motion patterns and analyzes accumulated data to make informed decisions.
This enables industries to establish predictive maintenance plans and helps designers optimize production line workflows.
Implementation Case
Automotive Sheet Metal Welding Quality
Problem: If welding is insufficient or defective, the joint may break or deform under stress, compromising structural stability and durability.
Solution: Comparing Current Signals for Automatic Welding Quality Assessment
Sheet metal welding involves automated welding robotic arms assembling various components into the car body.
In this project, the VMS-ML system was installed at the workstation, using a current clamp meter based on the characteristics of the welding robotic arm to calibrate current reference points.
The system quickly learns the correct manufacturing process standards and automatically identifies and tracks signals. By comparing current signals, it determines welding quality automatically.
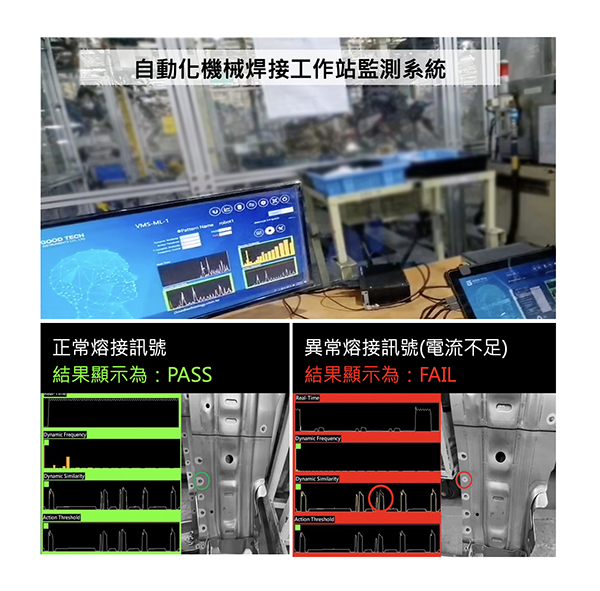
Benefits & Results:
The system detects abnormal signals in real-time and issues alerts to prevent human errors in judgment.
This helps users monitor the production line status and respond promptly, ultimately improving product quality.
Automated Spray Painting Robot Arm Monitoring
Reason for Implementation
Abnormal Movements Leading to Uneven Coating Thickness
The automated spray painting robotic arm is one of the core devices in modern industrial automation, widely used in automotive manufacturing, home appliance production, aerospace, and other industries.
However, when the robotic arm malfunctions, it can cause uneven coating thickness, negatively affecting product quality and production yield.
Common Issues in the Coating Process:
・Uneven paint adhesion (e.g., blotches, bubbles, sagging, or inconsistent thickness on the coated surface, affecting the final product’s appearance and durability).
・Insufficient robotic arm precision (deviation in motion trajectory or abnormal speed causing the coating thickness to fail to meet specifications).
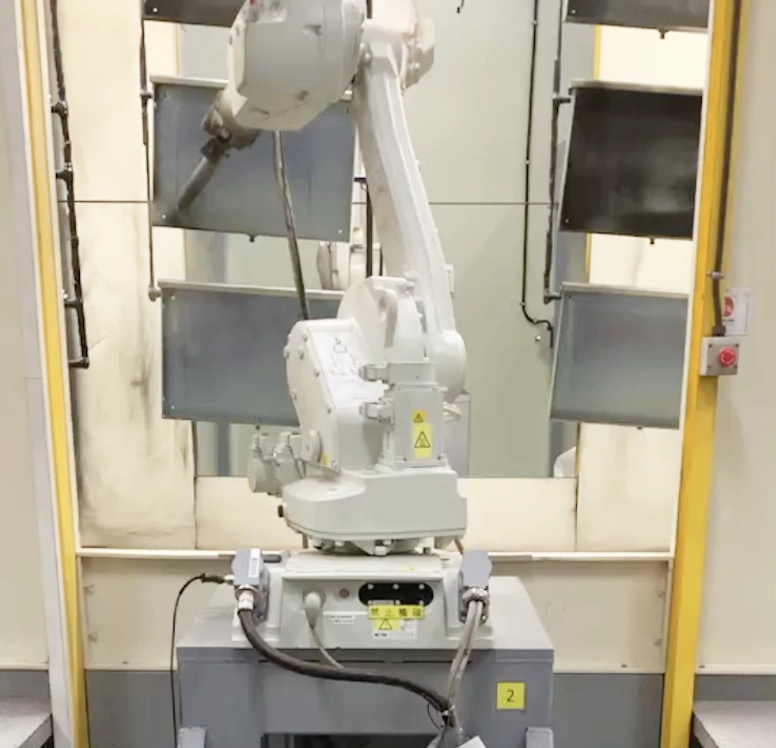
Implementation Challenges
Difficult to Detect the Exact Time of Arm Malfunctions?
The operation of automated spray painting robotic arms involves multiple motion parameters, and abnormalities may accumulate over time, making them difficult to detect in real-time.
Without instant monitoring and data analysis, abnormalities may only be noticed after they have already affected coating quality.
Implementation Method
Identify the Timing of Abnormalities to Ensure Smooth Operations
If the motion trajectory of the spray painting robotic arm deviates, the coating thickness may not meet expectations.
Therefore, by monitoring each movement standard, the system can track the arm’s operational status and prevent coating irregularities caused by abnormal vibrations.
Utilizing sensor data analysis and an anomaly warning system enhances the ability to detect equipment abnormalities, ensuring stable operation.
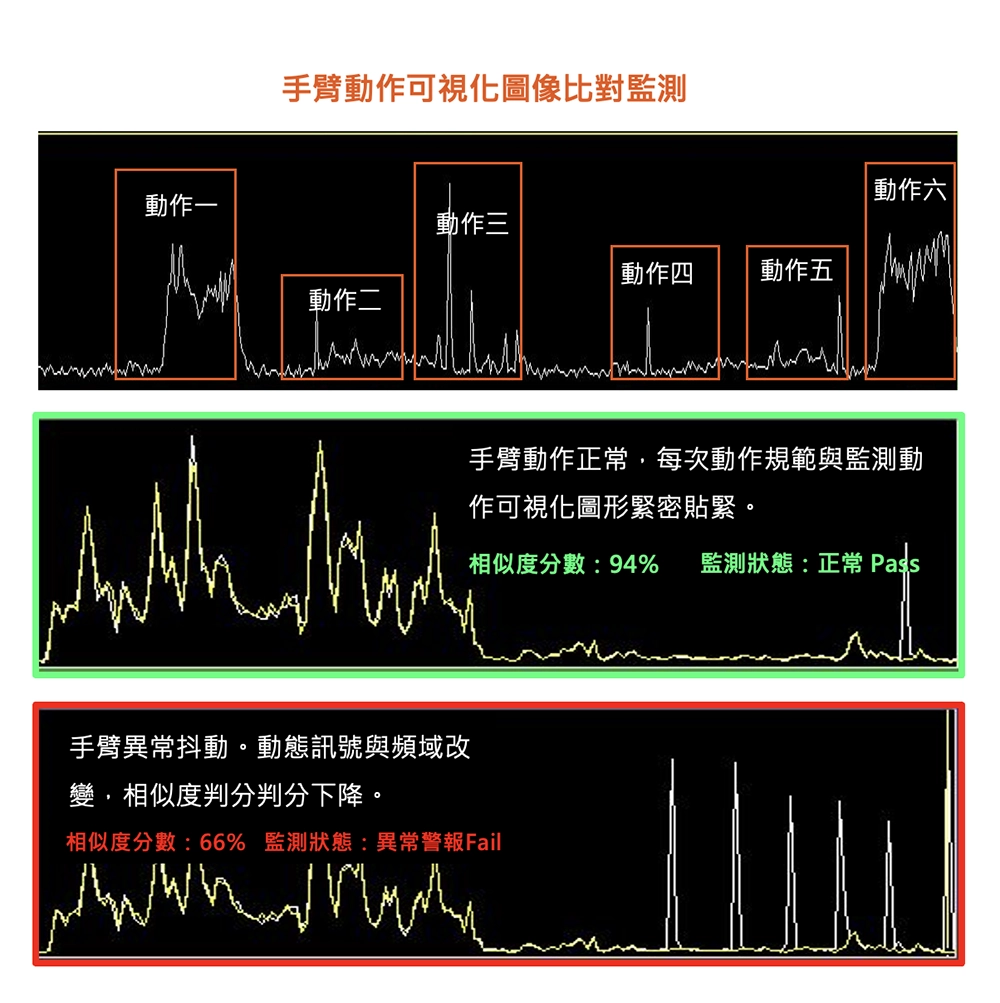
VMS®-ML Machine Learning Intelligent Monitoring System
The VMS®-ML Machine Learning Intelligent Monitoring System learns correct motion behaviors as a reference and monitors and diagnoses each movement.
It identifies which motion of the equipment experiences anomalies or instability, enabling proactive predictive maintenance.
By collecting health history trends, the system provides a data-driven reference for maintenance planning.
Implementation Case
Enhancing Work Safety and Ensuring Even Coating
Problem: The primary function of an automated spray painting robotic arm is to perform efficient and high-precision coating.
However, if the arm malfunctions, it may result in uneven coatings or missed spray areas.
Solution: Comparing Arm Motion Trajectories to Ensure Consistent Coating Quality
By installing sensors at the base of the robotic arm, the system learns the correct motion trajectory to ensure uniform coating quality.
Benefits & Results:
The VMS-ML system automates quality control, reducing workers’ exposure to hazardous chemicals during the painting process.
It precisely controls paint usage, reducing waste and emissions. Additionally, it ensures that the paint evenly covers all surfaces, preventing missed or excessive spraying.
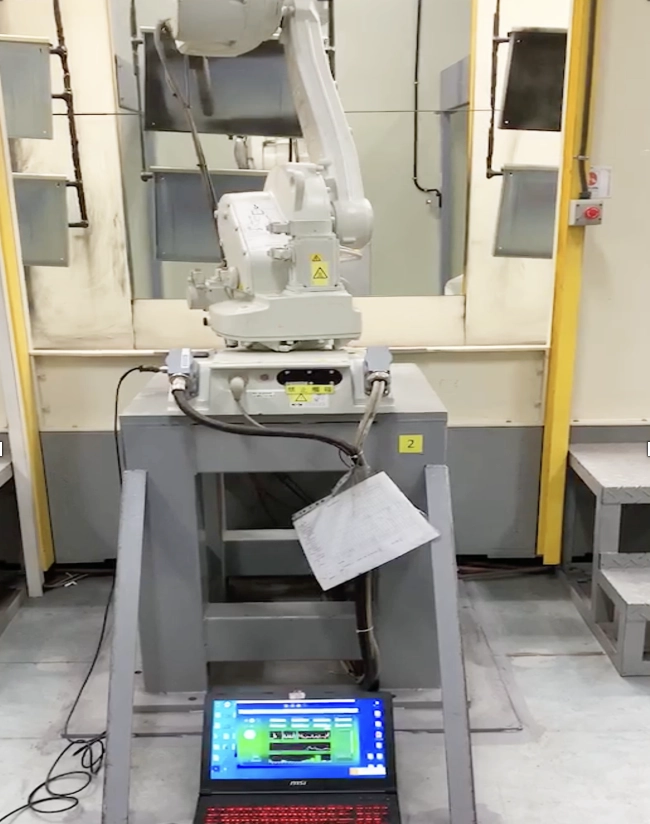
Large-Scale Handling Robot Arm Monitoring
Reason for Implementation
Arm Malfunctions Lead to Losses
Currently, more than 70% of global automotive manufacturing production lines use automated robotic arms.
These large-scale robotic arms perform tasks such as transportation, assembly, cleaning, and welding with high speed, safety, accuracy, and repeatability, handling heavy-duty work that is difficult for manual labor.
Issues When Robot Arm Malfunctions:
However, machines cannot communicate their issues on their own.
If the arm’s quality is not properly monitored, malfunctions such as collisions, mechanical damage, or unstable power supply can occur.
The arm may collide with other equipment or workpieces, causing damage or getting stuck, while power instability or outages may disrupt normal operations.
These issues not only increase repair or replacement costs but also lead to production downtime and economic losses, and in severe cases, may pose workplace safety risks.

Implementation Challenges
Large Amplitude During Start/Stop Leads to Misjudgment?
Large robotic arms have highly complex operational movements, and they often experience significant amplitude fluctuations during start and stop phases.
Moreover, normal and abnormal signals frequently overlap during operation. If abnormal signals are judged solely based on numerical thresholds, it may lead to false detections.
Implementation Method
Establish Standards, Automatically Track, Identify, and Evaluate
By utilizing the VMS®-ML Machine Learning Intelligent Monitoring System,
automated tracking and identification technology applies different learning standards based on various manufacturing modes, enabling fully customized threshold monitoring.
Additionally, the system automatically detects and identifies calibrated target signals in real-time monitoring.
Even if mechanical motion anomalies or external interference occur during the manufacturing process, the system can accurately determine whether the data signals belong to the same cycle and provide evaluation and results.
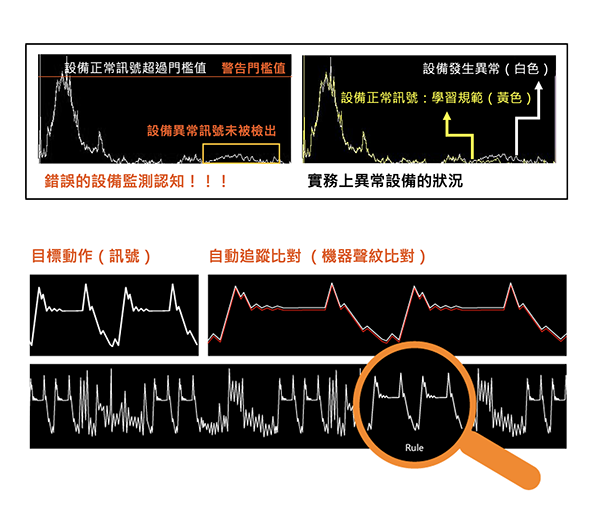
Implementation Challenges
Too Many Types of Large Robotic Arms, Making Monitoring Difficult?
Large robotic arms are responsible for various processes in automotive manufacturing, each requiring different monitoring specifications.
Additionally, multiple types of robotic arms may coexist on the same production line, requiring maintenance engineers to be proficient in multiple monitoring software systems.
Implementation Method
Flexible Application, One System for Multiple Devices
The VMS®-ML Machine Learning Intelligent Monitoring System is designed for various cyclic production equipment.
It supports multi-channel versions as needed, allowing a single system to monitor multiple devices.
The system employs algorithms to present key results in a simple red-yellow-green signal format along with a score.
Internally, it retains multiple data scores and characteristic maps while providing multiple configurable standards for user customization.
The operation is simple, and the results are easy to interpret, enabling engineers to start monitoring immediately after a brief training session.
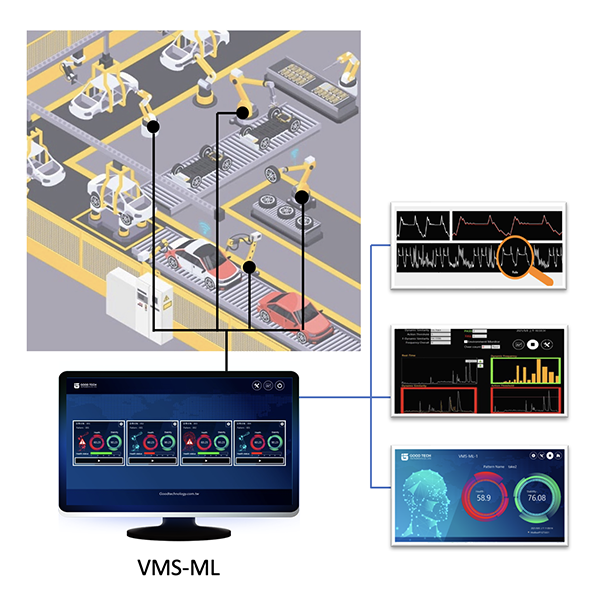
Implementation Challenges
How to Determine the Optimal Timing for Predictive Maintenance?
Large robotic arms must operate stably for extended periods while avoiding frequent failures or shutdowns.
The arm's operation should not pose a threat to operators or other equipment and must be equipped with appropriate safety devices and shutdown mechanisms.
Proper execution of predictive maintenance is crucial to ensuring equipment reliability and stability.
Additionally, determining the right maintenance timing and handling abnormalities is a key factor in maintaining production efficiency and quality.
Implementation Method
Making the Most Accurate Decisions to Optimize Workforce Allocation
The VMS®-ML Machine Learning Intelligent Monitoring System monitors different dynamic signals and learns their characteristics.
By continuously accumulating and comparing data and features, the system provides repair guidelines as a reference for maintenance scheduling.
Additionally, AI-driven predictive analysis estimates the remaining lifespan of equipment, allowing users to prepare for maintenance in advance.
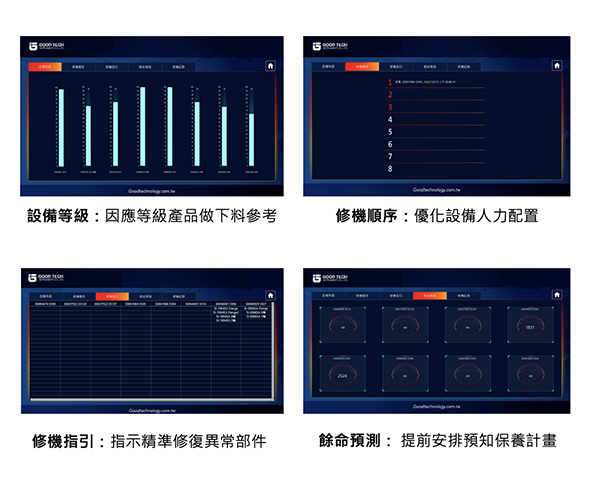
VMS®-ML Machine Learning Intelligent Monitoring System
Proper execution of predictive maintenance is a crucial factor in ensuring equipment reliability and stability.
Additionally, determining the right maintenance timing and handling abnormalities is essential for maintaining production efficiency and quality.
The VMS®-ML system dynamically monitors various mechanical equipment, learning operational patterns and making statistical analyses based on accumulated data.
Wafer Transfer Robot Arm Monitoring
Reason for Implementation
Even the slightest movement can affect wafer quality
Robotic arms play a crucial role in semiconductor wafer processing, handling tasks such as wafer processing and product transportation.
Even the slightest movement can impact wafer quality. For high-value products, customers place great importance on every stage of the manufacturing process,
ensuring product quality remains uncompromised throughout both production and transportation.
Challenges in Wafer Transfer Robot Arm Monitoring:
If the robotic arm malfunctions but remains operational, the risk of wafer damage is extremely high.
Due to the limited space in slot storage, any slight change in the arm’s posture may cause minor scratches or contact, which conventional systems may fail to accurately detect.
Currently, most wafer transfer equipment on the market struggles to precisely detect these minor anomalies.
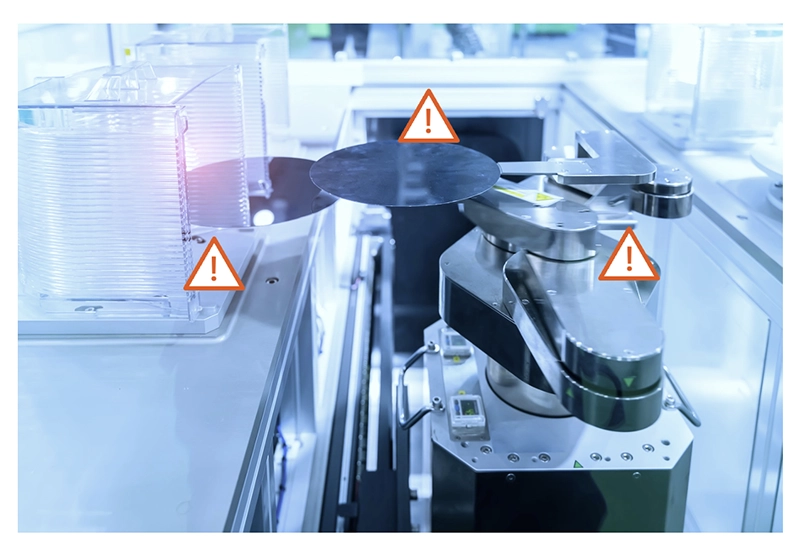
Implementation Challenges
Difficult to detect minor vibrations in robotic arms?
When a wafer transfer robotic arm experiences slight vibrations, it is difficult to detect anomalies in real-time, yet such vibrations can affect wafer positioning accuracy and production yield.
Potential causes of vibration include motor drive abnormalities, gearbox wear, control parameter deviations, external vibration interference, or bearing degradation.
Due to the small amplitude of these vibrations, traditional monitoring methods may struggle to detect and assess them in real-time.
Implementation Method
Continuous Cycle Run for Daily Operation Monitoring
The VMS®-ML Machine Learning Intelligent Monitoring System independently models and monitors each device to prevent discrepancies between individual units from affecting monitoring results.
Additionally, the system meticulously tracks and records every wafer transfer motion, both into and out of storage, in real-time.
Motion Detection Rate 100% ; Accuracy 100%
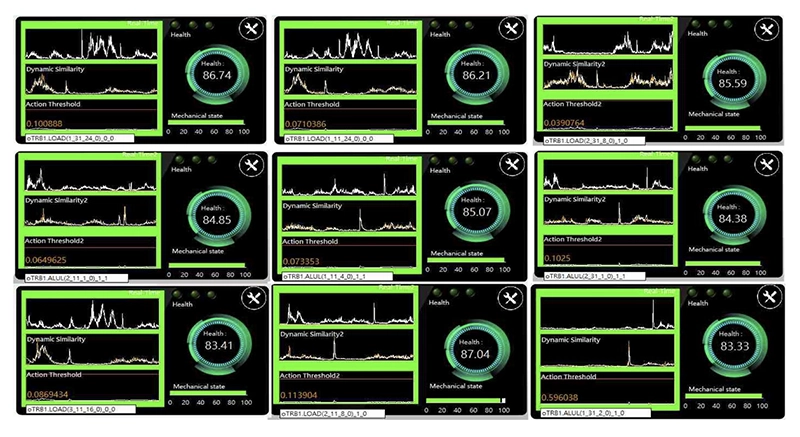
VMS®-ML Machine Learning Intelligent Monitoring System
The system precisely tracks and records each robotic arm’s wafer transfer motion into and out of storage in real-time.
It analyzes operating conditions, detects early signs of abnormalities, and instantly identifies issues, allowing for immediate shutdown to prevent wafer scratches.
Execution Process
Robotic Arm Smart Monitoring Solution Workflow
New Feature Launch
Repair Guidance & Remaining Life Prediction

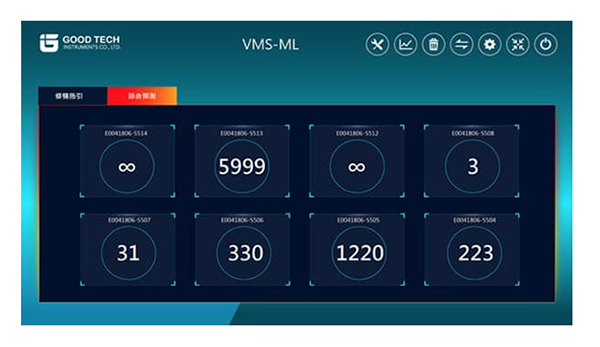
Beyond equipment quality status, users want to know: Where is the abnormality? How much longer can it be used?
By integrating various sensors with a monitoring system, data from the measured object is collected (data measurement).
The system selects the necessary data (data selection), removes noise (data preprocessing),
and then extracts relevant features. By analyzing abnormal characteristics and collecting trends,
it determines the location of equipment damage and predicts its remaining lifespan.
Repair Guidance: Identifies which axis of the robotic arm is experiencing abnormalities, reducing repair and inspection time.
(VMS-PH can be used to detect the cause of the abnormality.)
Remaining Life Prediction: Provides a clearer time frame based on predictive analysis, allowing for early maintenance planning based on equipment lifespan.
Implementation Benefits
Optimized Decision-Making, Problem Solving
Prevent Production Line Downtime and Increase Operation Time
By implementing predictive maintenance planning, production lines can be effectively managed to avoid unplanned equipment maintenance and waiting time for parts.
This allows for more efficient execution of production tasks, extending usable operation time by up to 23%.
Benefit: Efficiently Scheduling Production Lines
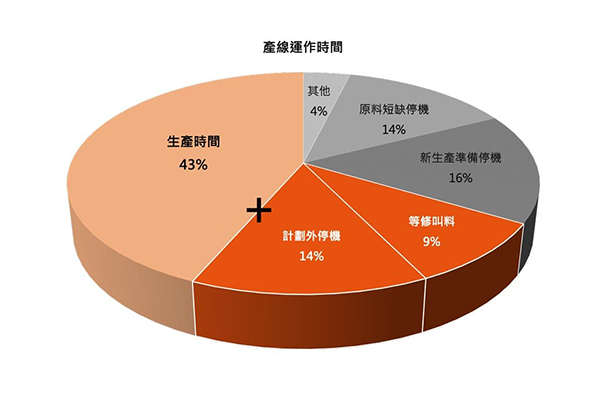
Early Anomaly Detection to Prevent Major Losses
By predicting equipment failures in advance, maintenance and material procurement can be initiated as soon as failure indicators are detected.
This significantly reduces the risk of unexpected shutdowns and severe equipment damage by up to 95%.
Benefit: Reducing Wear and Tear Risks
Extend the Usable Life of Equipment
By performing maintenance based on the actual health status of equipment, its lifespan can be maximized while avoiding unnecessary maintenance.
This approach improves equipment efficiency by approximately 53%.
Benefit: Avoiding Excessive Maintenance