How to Prevent Welding Defects Caused by Excessive Current in Robotic Arms?
Case | How to Prevent Welding Defects Caused by Excessive Current in Robotic Arms?The principle of welding robotic arms is to introduce high-voltage current into the welding area, generating heat to fuse metal materials. If excessive current is applied or the positioning is incorrect, welding defects may occur. How can we prevent these issues?
Operating Principle of Welding Robotic Arms
The welding robotic arm operates by introducing high-voltage current into the welding area,
generating heat to fuse metal materials together. This process requires an electrically conductive welding rod
to transmit current, with heat generation controlled by adjusting the current intensity and duration.
The arm is designed to provide stable positioning and movement, ensuring welding accuracy and consistency.
Automated welding robotic arms function based on user-programmed steps, including positioning, time parameters,
motion parameters, and welding settings. These arms execute automated operations accordingly. To accommodate
various manufacturing processes while saving space, welding robotic arms feature multi-axis joints for optimal
movement flexibility in complex environments. Traditional welding techniques have now evolved into
automated robotic welding, offering greater stability and efficiency.
In the Industry 4.0 era of smart manufacturing, automated welding robotic arms have largely replaced human workers
in hazardous welding tasks. As technology advances, robotic welding has been widely adopted in industries
such as automotive and engineering machinery. But how can welding quality be managed using scientific data?
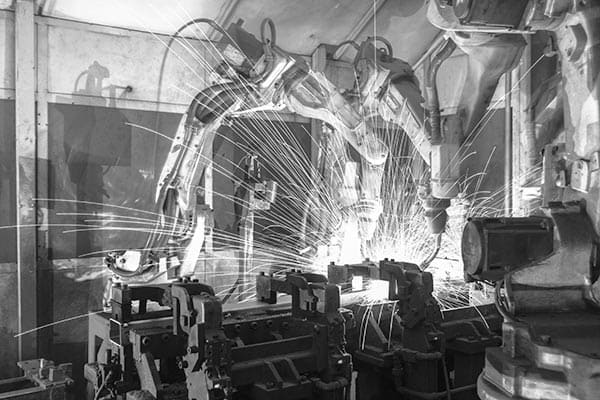
Common Issues in Welding Robotic Arm Processes
The welding robotic arm process often encounters the following challenges:
Welding Defects: If the welding current is too high or too low, or if the welding position is incorrect, defects may occur.
Damaged Welding Arm: Welding robotic arms endure high temperatures and pressure, making them prone to damage over time.
Power Failures: Power failures can disrupt welding operations, requiring adjustments and recalibrations.
Control System Malfunctions: If the control system malfunctions, welding accuracy and continuity may be compromised.
Worn Welding Rods: Prolonged use can cause welding rods to wear out, necessitating replacements.
These issues can be proactively prevented through a monitoring system, coupled with proper maintenance
and regular inspections to ensure the normal operation of welding robotic arms.
Solution and Monitoring Explanation
VMS-ML Machine Learning Intelligent Monitoring System
By utilizing the VMS-ML machine learning intelligent monitoring system and installing current characteristic
sensors, the system performs real-time monitoring of spot welding operations. Through software-based time-domain signals,
frequency-domain signals, Fast Fourier Transform (FFT), real-time surge analysis, and instant comparison techniques,
the system detects normal and abnormal welding behaviors. This enables real-time monitoring and predictive maintenance,
ensuring high product yield and maintaining automation efficiency.
Measurement Status
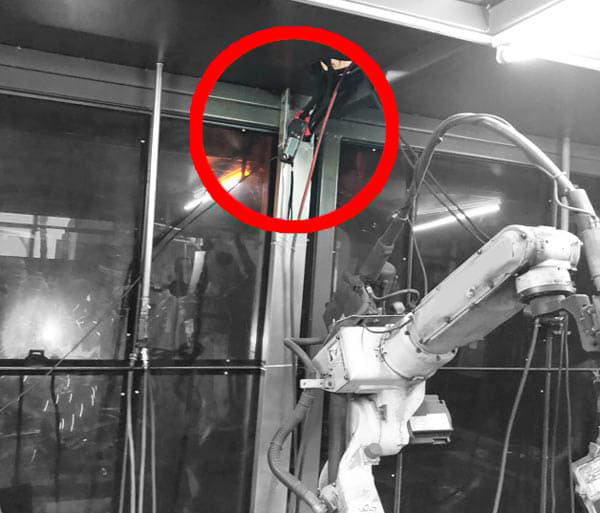
Sensor Selection:
Select sensors based on process characteristics. Since welding robotic arms use current to generate heat,
a current sensor is used for monitoring.
Current Measurement Location:
The current clamp meter is externally connected to the DC output line of the spot welding current,
directly capturing the welding current signal for monitoring. The system can learn designated spot welding
current signals (clamp meter connected to the positive terminal).
System Measurement Explanation:
The VMS-ML Machine Learning Intelligent Monitoring System uses a non-intrusive measurement method, enabling
plug-and-play monitoring. By learning the correct signal, the system automatically classifies signal types
and captures target signals in real time.
Welding Robotic Arm Measurement Results
Monitoring Status: Normal Pass
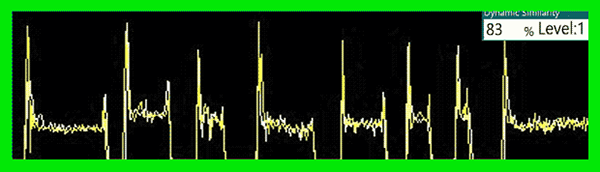
The system recognizes and monitors the stability of welding current signals.
Similarity Score: 83%
Monitoring Status: Abnormal Alarm Fail
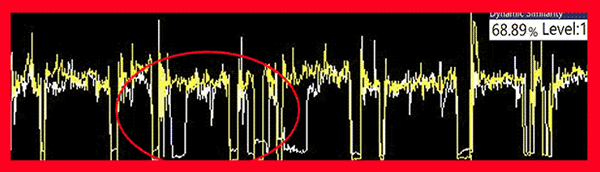
The system detects abnormal welding current signals, leading to a lower similarity score.
Similarity Score: 69%
Features and Trends
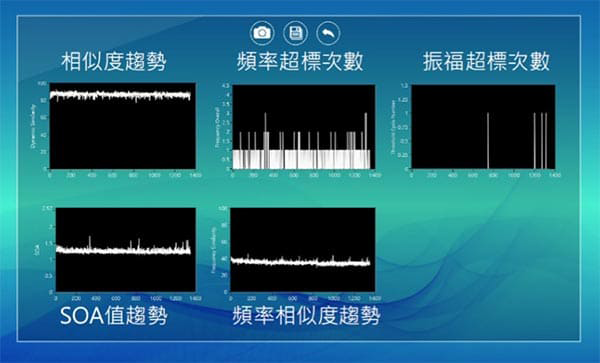
Measurement results can be used for future AI-driven smart manufacturing management.
Measurement Conclusion
Using the VMS-ML Machine Learning Intelligent Monitoring System, correct motion behaviors are learned as benchmarks, and process current thresholds are established for comparison and monitoring. A single instance of abnormal detection can clearly distinguish signal deviations, while long-term monitoring enables predictive maintenance by identifying machine operation status and ensuring product quality. A drop in similarity score indicates unstable current and potential product defects.
The VMS-ML Machine Learning Intelligent Monitoring System enables real-time monitoring and diagnostics of individual welding actions. By identifying abnormal or unstable conditions early, predictive maintenance can be performed, preventing unexpected failures.
VMS-ML Machine Learning Intelligent Monitoring System