How to Monitor the Operational Quality of Wafer Dicing Machines?
Case|How to Monitor the Operational Quality of Wafer Dicing Machines?The wafer dicing machine is a critical piece of equipment in semiconductor manufacturing. Its quality significantly impacts the entire process and the final product performance. How can we ensure the stability of the machine's operational quality?
Wafer Dicing Machine
The Relationship Between Wafer Dicing Machine Stability and the Manufacturing Process The stability of a wafer dicing machine is directly related to the stability of the entire manufacturing process. A high-quality dicing machine must ensure cutting precision and consistency, achieving higher cutting accuracy to meet the required component dimensions and shapes. A high-performance wafer dicing machine can improve process speed, thereby enhancing production efficiency, which is crucial for large-scale manufacturing and tight production schedules. Therefore, if the cutting machine's efficiency is subpar, it may become a bottleneck in the entire process, affecting overall production capacity. By monitoring the operational movements of the dicing machine, we can ensure its quality, further achieving consistent manufacturing standards, reducing process variability, and improving process stability.
Monitoring Description
VMS-ML Machine Learning Intelligent Monitoring System
The system captures vibration signals synchronized with key operational actions such as spindle acceleration, wafer dicing, and other mechanical movements. It performs standardized learning under optimal conditions and evaluates operations by comparing them with predefined benchmarks. Through this comparison, it identifies repetitive actions to achieve mechanical operation quality monitoring and ensure process stability.
Measurement Conditions
Specification 1: Spindle Startup Inspection
Monitoring Items:
# Air-floating spindle operation quality
# Tool holder and thread quality
# Tool and protective cover fastening quality

Inspection Results
Spindle Startup Identification and Inspection
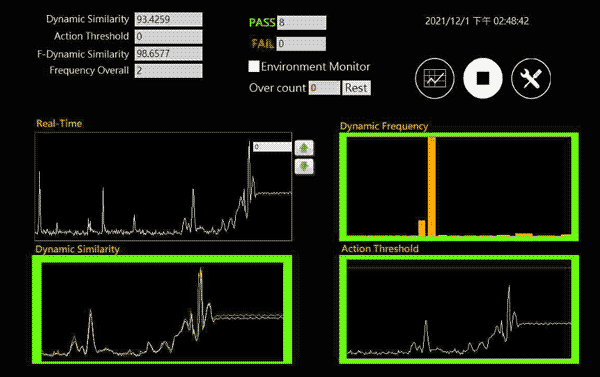
Automatic Identification Successful, Inspection Result: Pass (93.43%)
Spindle Startup Identification and Inspection
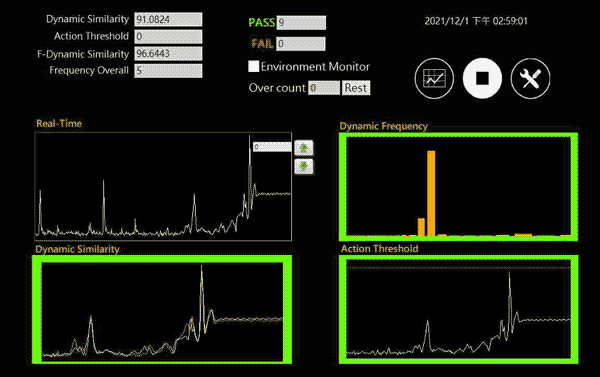
Automatic Identification Successful, Inspection Result: Pass (91.08%)
Specification 2: Wafer Cutting Process
Monitoring Items:
# Z-axis movement and positioning quality
(Z-axis servo motor and driver, Z-axis lead screw, and slide components)
# Y-axis movement and positioning quality
(Y-axis servo motor and driver, Y-axis lead screw, and slide components)
# X-axis movement (cutting) quality
(X-axis servo motor and driver, X-axis lead screw, and slide components)

Wafer Cutting Specification Recognition
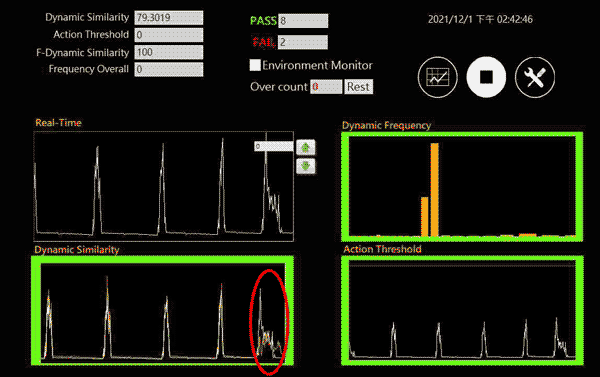
Cutting First Side: Pass (79.3%)
Cutting Second Side [Rotation]
![Cutting Second Side [Rotation]: Pass (86.71%)](../img/case/case-ds/case-ds-06.webp)
Cutting Second Side [Rotation]: Pass (86.71%)
The vibration dynamics of directional cutting are similar, and the differing parts at the end (marked in red) can be filtered out (a standard built-in software feature). It is recommended to use this as an actual monitoring specification.
Specification 3: Wafer Cutting
Monitoring Items:
# Quality confirmation before and after lead screw replacement
Before Lead Screw Replacement
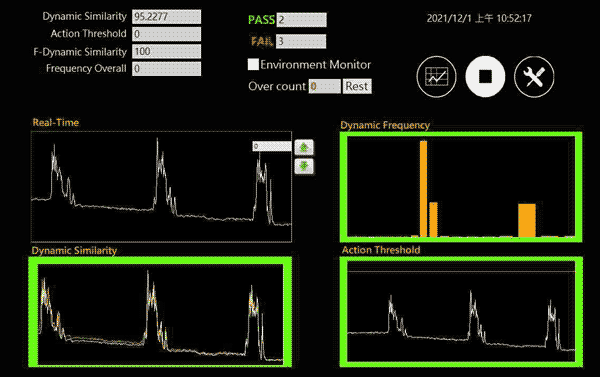
Automatic recognition success, test result: Pass (95.23%)
After Lead Screw Replacement
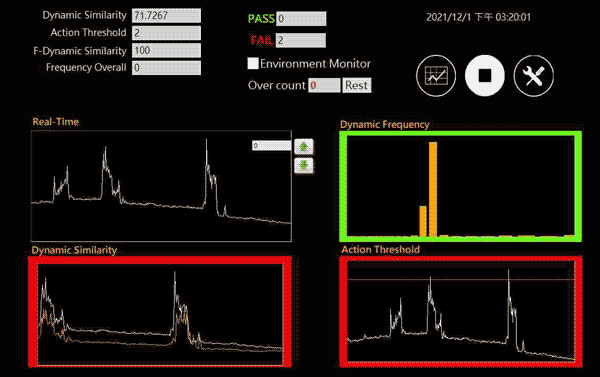
Automatic recognition success but test result: Fail (71.72%)
(indicating excessive movement variation of the lead screw)
Equipment health check result: Fail (indicating significant movement deviation)
Measurement Conclusion
1. The upper structural model can measure most of the equipment vibrations, and the monitoring system can clearly recognize each operation.
2. The vibration dynamics after wafer cutting and rotation are similar, allowing the same specification to be used for testing.
3. Significant differences in actions were observed before and after lead screw replacement, with a large drop in the VMS-ML score evaluation.
4. Slight cutting axis vibration resulted in a score drop; after adjustment and reset, the score returned to pre-vibration levels.
By establishing simple health standards, the system learns operational actions and makes analytical judgments based on accumulated data. Users can then develop predictive maintenance plans and assist designers in optimizing production line workflows.
VMS-ML Machine Learning Monitoring System