How to Prevent PCB Cutting Defects from Damaging Products?
Case|How to Prevent PCB Cutting Defects from Damaging Products?PCB cutting machines are used in the electronics manufacturing process to cut PCB boards. If the cutting path extends beyond the intended area and reaches the copper layer, it can negatively impact the quality and performance of the PCB. How can this be prevented?
PCB Cutting Machines
PCB (Printed Circuit Board) cutting machines are used in the electronics manufacturing process to cut PCB panels.
PCBs are the core components of modern electronic products, filled with electronic components and connecting circuits, enabling the proper functioning of electronic devices.
The main function of a cutting machine is to cut large PCB panels into the required sizes for further processing and assembly.
The relationship between the cutting process and current consumption is influenced by a combination of complex factors.
To ensure cutting stability and quality, operators must select appropriate settings based on different PCB materials, cutting parameters, and machine models to keep current consumption within a reasonable range.
If the cutting path extends beyond the intended area and reaches the copper layer, it may negatively impact the PCB’s quality and performance.
For example, it can create an electrical connection between copper layers, leading to short circuits.
This could result in circuit board failure, product malfunction, and potential damage to other electronic components.
Key Monitoring Points:
1. Cutting precision is crucial for product quality.
It is essential to monitor the cutting accuracy to ensure precise dimensions and prevent assembly issues in subsequent processes.
2. Cutting pressure and speed must be adjusted according to different PCB materials and designs.
Excessive pressure or high speed can lead to uneven cuts or board damage.
3. The cutting tools of the machine require regular inspection and maintenance.
Ensuring blade sharpness and stability helps avoid poor cutting quality or blade breakage issues.
Solutions and Monitoring
VMS-ML Machine Learning Intelligent Monitoring System
The VMS-ML machine learning intelligent monitoring system is used to learn and establish monitoring standards based on the cutting program sequences of good (acceptable) products.
Any deviations beyond the established standards are identified as defects.
Current sensors are selected to monitor the current signals generated during spindle operation, providing real-time insights and early detection of anomalies.
Measurement Conditions
Measurement Condition 1: Perfect Edge Cutting
Result: Current recognition successful, evaluation score: 95.4.
Status: PASS
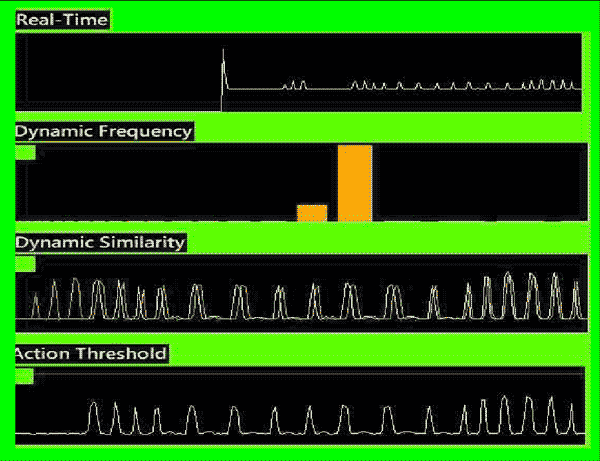
Monitoring Status: Normal Pass
Measurement Condition 2: Cutting Path Extended to Copper Layer
Result: Current recognition successful, evaluation score: 74.3.
Status: FAIL
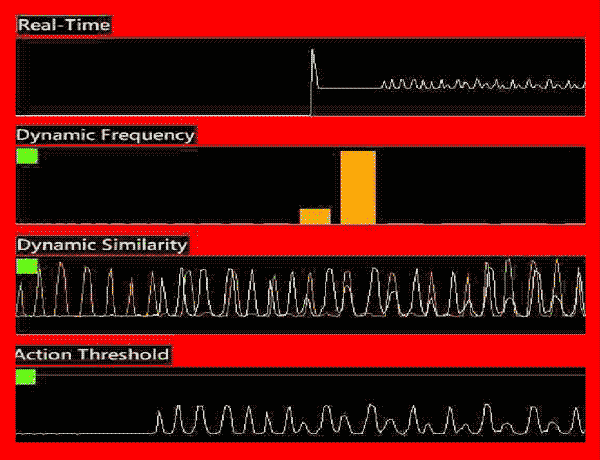
Monitoring Status: Abnormal FAIL
Detailed Waveform Signal Comparison
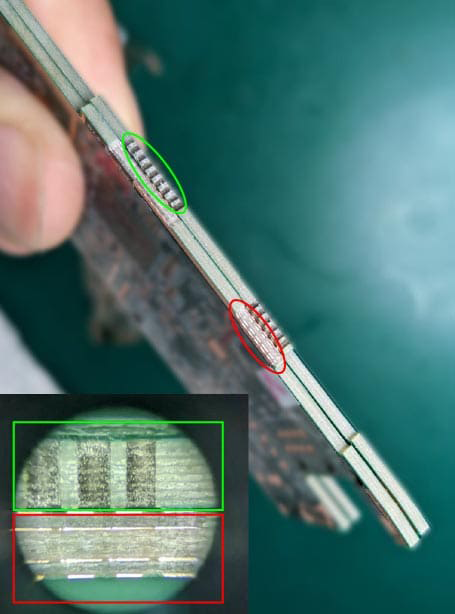
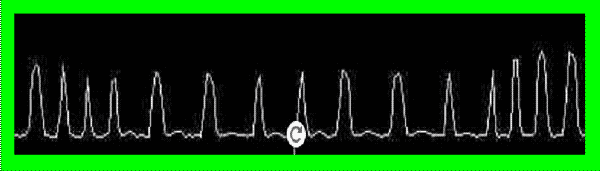
Normal Current Signal
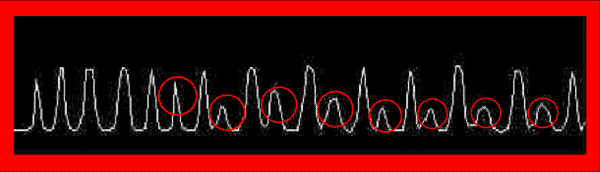
Abnormal Current Signal
# The current signal shows a significant difference when cutting into the copper layer, which can be used as an anomaly feature.
Measurement Conclusion
Using the VMS-ML Machine Learning Intelligent Monitoring System to learn the correct cutting actions as a standard,
combined with process experience to establish current monitoring specifications for process management.
1. The quality monitoring results of the PCB cutting machine spindle current show that VMS-ML can successfully detect abnormal spindle current variations.
2. Since each machine spindle condition varies, monitoring standards can be established based on equipment and product process part numbers in the future.