Common Issues in the Packaging Industry?
Application Field |Packaging IndustryThe packaging process involves intricate details and high-precision, highly automated equipment. The main challenge lies in managing equipment and ensuring process quality.
Execution Motivation
Common Issues in the Packaging Industry?
Increasing Complexity and Higher Precision Requirements
The semiconductor manufacturing process consists of wafer fabrication (Front-End) and packaging & testing (Back-End). With the advancement of packaging technologies, an intermediate processing stage called Middle-End has emerged between wafer fabrication and packaging. As chip sizes approach physical limits, advancements in front-end processing are slowing, shifting focus to back-end packaging and testing.
According to Yole Développement’s industry analysis report, traditional packaging still dominates the market. However, with increasing demand for advanced semiconductor chips and complex packaging structures, the growth of advanced packaging will become a major driver. By 2027, the advanced packaging market is expected to reach $65 billion, with a compound annual growth rate of 10%, and is projected to surpass 50% market share.
Packaging technology is categorized into traditional and advanced packaging. Traditional packaging includes key steps such as back grinding, wafer dicing, die attachment, wire bonding, molding, electroplating, lead trimming/forming, and final testing. Advanced packaging technologies, such as **2.5D, 3D, and CoWoS**, utilize stacking methods to maximize space efficiency. This integration enhances system performance, achieves a smaller packaging footprint, and improves thermal management, making the process more complex and requiring greater precision.
Execution Challenges
Factors Affecting Packaging Quality?
Equipment, Process, and Energy Consumption Management
The semiconductor packaging industry involves intricate details at every stage, requiring high-precision and highly automated equipment. Ensuring equipment quality is key to guaranteeing product quality. Management areas include **equipment quality management, process control, and energy consumption management.** Any deviation in process quality can lead to product failure. Additionally, equipment performance impacts process efficiency and energy usage. Therefore, manufacturers must closely monitor equipment status to ensure high-quality, high-performance packaging production.
Equipment Quality Management in the Packaging Industry
Key equipment includes **wire bonders, die bonders, die saws, laser cutting machines,** etc. These machines contain precision components such as **drive motors, ball screws, robotic arms, bonding heads, temperature control systems, conveyor systems, operational platforms, and environmental control systems.** Adhering to high-precision and high-reliability standards is crucial to achieving high packaging yields.
Process Quality Management in Packaging
Even when process equipment operates in optimal condition, human input errors, environmental factors, or inconsistencies in component operation can lead to defects. Minor errors in alignment or timing can result in **wafer breakage, product failure, delayed deliveries, premature equipment wear,** and other issues.
Energy Consumption Management in Packaging
With the growing importance of ESG in the semiconductor industry, reducing carbon emissions is a key priority. Upstream suppliers demand lower-carbon products, while downstream manufacturers implement **green circular optimization solutions** to manage equipment energy consumption. This not only optimizes equipment efficiency and reduces emissions but also enhances factory utilization rates and cost savings.
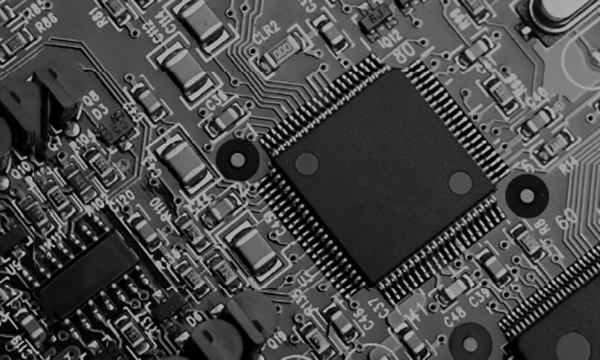
Execution Goals
Predictive Diagnostics for Packaging Equipment
# Ensure Equipment Quality to Prevent Defective Products
**Wafer-level equipment (LG, DS):** High dependency on production capacity—unexpected downtime affects scheduling. Monitoring equipment health is critical! **Die-level equipment (DB, WB):** Using real-time process monitoring as an online quality control solution. Immediate alerts on equipment abnormalities prevent mass production of defective products, protecting customer interests.
# Precision Maintenance Over Trial-and-Error, Reducing Labor Costs
With increasing automation and a shift toward higher machine-to-operator ratios, predictive maintenance must replace traditional scheduled maintenance. Optimized equipment management allows precise maintenance actions, minimizing **spare parts waiting time, reducing replacement errors,** and improving machine recovery efficiency.
# Prevent Human Errors and Control Environmental Factors
Even with well-maintained equipment, proper operation is essential. **Error-proofing mechanisms** must be in place to prevent mistakes due to human input. Environmental factors such as **temperature, humidity, pressure, and particle contamination** must be monitored and controlled using data-driven threshold settings to optimize process protection.
# Optimize Decision-Making to Reduce Energy Consumption and Carbon Emissions
Monitoring factory energy consumption provides insights into equipment usage patterns, enabling **maximized efficiency, reduced idle time, optimized production line utilization,** and data-driven sustainability initiatives such as **carbon management, cost-benefit analysis, and green energy programs.**
Execution Strategy
Real-Time Quality Monitoring & Decision Optimization
For managing various cyclic equipment, the **VMS-ML Machine Learning Intelligent Monitoring System** learns correct process dynamics and employs automated tracking technology to establish customized monitoring models. The system achieves a **target tracking accuracy rate of less than 0.1% false positives.**
By continuously monitoring characteristic value trends, users can analyze the aging process of machines, define future maintenance plans based on trend data, and implement secondary threshold management and AI training to predict potential variations. Additionally, by collecting various steady-state physical and machine signal data, **firmware-level and system-level edge computing** converts large amounts of physical data into actionable monitoring parameters. This enables **real-time quality monitoring and optimized decision-making** through threshold settings.
VMS-ML Robotic Arm Monitoring System
Support
Other Semiconductor-Related Fields